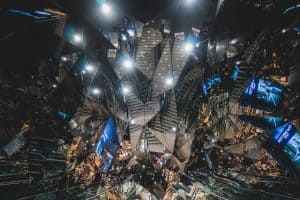
Using IIoT and AI improves decision-making and management by blending internal and external data into predictions, insights, and choices to guide operational actions.
With the emergence of the Industrial Internet of Things (IIOT) and artificial intelligence (AI), a new type of factory is possible – driven by data and empowered by digital algorithms and analytics. This digital factory is more than just automation; it’s an intelligent factory that enables a new operating model. Gone will be the days of long-lead-time demand forecasting and production scheduling. Instead, demand forecasting and production scheduling will be driven by near real-time requirements for product features, inventory management, assembly, finishing, and delivery.
The new digital operating model of the intelligent factory will remove human bottlenecks from both production and decision-making. Digital technologies can be deployed in modular architectures that easily connect within the factory and with other collaborating organizations. This system-of-systems approach makes sequential deployment possible, while recognizing the interconnectedness of each of the system components. This approach accelerates the transition of successful pilots to full-scale production and increases learning across the multiple components in IIOT + AI installations. Ultimately, a factory empowered by IIoT + AI reduces the guesswork of decision-making and management by supporting them with software and analytics that blend internal and external data into predictions, insights, and choices that can guide operational actions.
Preparation is required to take advantage of the power of the digital operating model, including these eight fundamental steps that companies need to consider.
1) Establish Clear Goals: Understand what digital transformation can do for your company’s operations. How might software-defined manufacturing systems, which decouple hardware and software from processes, change individual operations, and overall system performance? Once you understand how your manufacturing operations can be transformed, you can then pilot projects sequentially.
2) Develop Industrial Protocol Convergence Strategy: Determine if your operating environment is supported by many different protocols and communication networks. To develop modular systems, you must standardize handoffs within the operational environment, ultimately pursuing open standards for long-term sustainability.
3) Make Databases Ready for AI: Identify the type of data (e.g., audio, video, time-series, and cost) that will be input into AI algorithms to recognize patterns and make recommendations. It is important to decide upfront where AI algorithms will run – from the edge to the cloud – and how this will impact the data that is processed, communicated, and stored. This will require a centralized view of the company’s data structures and locations.
4) Understand Software Stack Tradeoffs: Consider the software tradeoffs associated with the architecture you deploy, like whether you will make or buy the AI software and whether you will depend on proprietary or open-source software. A final tradeoff is using a monolithic system or adopting a modular system. Companies making these choices need to balance their unique needs and existing systems with the ability to easily upgrade as underlying technologies supporting IIOT + AI evolve.
5) Map Out Hardware Deployment Model: Ensure the IIOT sensors you’re deploying into the operating environment won’t overwhelm your compute environment. Because of potential overload, companies need to determine where to process data. Many companies are finding large volumes of data output is better accommodated by edge computing – compute that is located where the data is being created at the device level.
6) Take a Close Look at Data Security: Evaluate if increasing the number of machines connecting to the network and simultaneously transmitting data will increase your cyberattack surface. To avoid this risk, companies need to identify mission-critical data and treat it accordingly with on-premise compute rather than sending it to a third-party cloud. Additionally, companies need to identify when and how to encrypt data to make it more difficult for hackers to compromise systems.
7) Explore Different Analytics Models: Investigate the various types of AI models you will need to deploy to address different situations. Do you need to train a model using existing data (machine learning), or do you need to have the model identify relationships based on emerging data (deep learning)? To answer these questions, you need to determine what digital models you have of the physical world and what data is available. Once again, trade-off decisions need to be made.
8) Act on the Analytics: Plan how the outputs from your IIOT + AI components will drive the decisions and actions needed to fully realize a new digital operating model. Building trust among workers, managers, and leaders of these digital systems is critical. Data analytics without action are meaningless.
Intel has been practicing these eight fundamentals beginning nearly three decades ago. We have had to balance data generation, compute location, and communications bandwidth, relying more and more on edge computing approaches. We are using AI to reduce the burden of data analytics to create near real-time insights that drive decisions and action. So, we have learned a thing or two along the way.
Because of our experience, many of the tools we are developing focus on interoperability, modularity, and open-source strategies. While these tools will make it easier for companies to accelerate their digital transformation journey, companies still face choices about what to deploy, how to deploy it, and most importantly, how to achieve sustainable digital operations.
Learn more about these key considerations in Intel’s new eBook: Eight Key Considerations When Implementing AI In Manufacturing.
Learn more about the technologies that are building the future of industry at the online Intel Industrial Summit 2020, September 23-24th. Register now