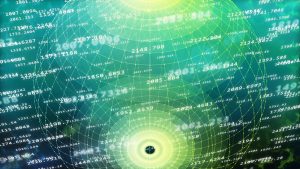
Multidimensional data observability can help enterprises observe and optimize a wide range of complex data systems, technologies, and use cases from a single unified view.
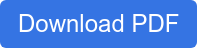
Can a digital payment processing startup that serves 350+ million customers, across 10 million retail stores, save over $5MM in recurring annual costs, while scaling their operations rapidly?
Yes. A data observability solution from Acceldata helped PhonePe, a WalMart subsidiary, reduce data warehouse costs by 65% as they rapidly increased their data infrastructure from 70 to 1,500 Hadoop nodes.
What’s more? With Acceldata Pulse, PhonePe maintained 99.97% availability across their data infrastructure and significantly minimized daily data emergencies.
“Acceldata supports our hyper-growth and helps us manage one of the world’s largest instant payment systems.” says Burzin Engineer, the Chief Reliability Officer at PhonePe (WalMart), “PhonePe’s biggest-ever data infrastructure initiative wouldn’t have been possible without Acceldata.”
That’s the happy outcome of this story, and in this article, we will define data observability, show you how it can deliver value to your organization, and discuss the eight steps required to implement it.
See also: Data Observability Resources
First, though, it’s important to understand that increasing data complexity is a big problem for enterprises such as PhonePe. In fact, data complexity is a growing problem for any data-driven company, and it can undermine the very value data is supposed to bring to the business.
The emerging practice of data observability can bring order to the chaos of data complexity by allowing teams to monitor data infrastructure and detect issues that might cause outages or constrain performance.
What Is Data Observability?
Data observability can be defined as the ability of an organization to completely understand the health of its data. Put another way, it is a systematic solution to the problem of data complexity. It monitors and correlates data workload events across application, data, and infrastructure layers to resolve issues in production analytics and AI workloads.
As the PhonePe example illustrates, data complexity is a huge issue, and it’s only getting bigger. Exploding data supply and demand are pushing modern data pipelines to their breaking points. Data teams are struggling to keep up.
By implementing data observability tools, organizations can expect to reduce and even prevent downtime. It gives them the ability to keep tabs on the health of data systems by monitoring, tracking, and troubleshooting incidents that can result in data downtime. And like PhonePe story, it can help enterprises save millions in annual data infrastructure costs.
Data observability solutions can use technology like automation and artificial intelligence to correlate alerts across an organization’s data infrastructure. They can pinpoint issues to help data teams solve problems, scale, and meet expectations.
Multidimensional Data Observability: Addressing Requirements at Every Layer of the Data Stack
In order to be effective, data observability solutions must address specific requirements at every layer of the enterprise data stack. This includes the following:
- At the infrastructure layer, they can help engineers avoid operational issues, performance bottlenecks, and system outages.
- At the data layer, they ensure data timeliness and quality stays in line with service-level agreements (SLAs).
- At the pipeline layer they can see data flowing through the pipeline, avoid blockage and optimize pipelines for better performance.
Simply put, data observability tools can empower data teams and business executives. They have multiple uses throughout your organization, helping automate routine tasks, streamline processes, and improve decision making.
To further illustrate how data observability can be used throughout an enterprise, let’s look at a few examples.
- DevOps, platform, and site reliability engineers can use it to manage infrastructure performance by configuring observability monitors for memory availability, CPU/storage consumption, and cluster/node status.
- Data architects and engineers can glean recommendations on how to tune the performance of the data pipeline to ensure timely, high-quality, and reliable data is delivered to consumers.
- Line of business and IT leaders can manage the business of data analytics more effectively for cost modelling and chargeback purposes.
How to Put Multidimensional Data Observability to Work
The three most common environments where data observability offers the greatest value include: on-premises Hadoop, hybrid cloud, and multi-cloud.
On-Premises Hadoop
Hadoop data lakes tend to persist in large on-premise environments, making them hard to manage. To address this, some data teams still run analytics and struggle to maintain performance levels across the many Apache open source components. Data observability is a useful alternative, helping data teams improve performance, reliability, and scalability.
Hybrid Cloud
To simplify the administrative work associated with managing converging data warehouses and data lakes, enterprises often adopt cloud data platforms. When they do, they need data observability to monitor cloud platform performance and reduce the risk of computing cost overruns.
Multi-Cloud
Many enterprise environments have multiple cloud service providers to allow them to optimize their workloads and meet specialized requirements. Data observability provides a way to help them oversee these topologies and keep data pipelines efficient and effective.
8 Steps to Putting Data Observability to Work
Setting up a data observability program is quite similar to setting up any new system. The more methodical you are, the better your chances of success become. Here are 8 steps to decrease complexity in your data stack:
1. Gather Requirements
A holistic approach is necessary to maximize the value of data observability. Make sure you have your users, use cases, technology components, and pipeline requirements covered across all the layers. Make sure to document your requirements, accounting for potential future needs.
2. Prioritize
Identify the requirements that are causing the most problems and can be most easily fixed. The most “painful” requirements can be determined by their impact on business metrics, while the most “fixable” are those that offer the best benefit at the lowest risk.
3. Scope Projects
Once the highest-priority requirements have been identified, the team needs can begin scoping them out. It’s best to start with small, achievable projects to demonstrate value to the organization, and then build on that momentum.
4. Assemble Your Team
Find cross-functional team members to drive the program. For a first project, select the team carefully, identifying those with the right technical and business knowledge.
5. Select Tool(s)
Evaluate the available solutions based on a few key criteria: sufficient visibility into the current and near-term future components of each layer, the level of customization required, training time required, and its ability to replace other tools in your environment.
6. Execute and Iterate
Start the execution of your project. It’s important to measure the success of each project, so success can be demonstrated, progress can be evaluated, and adjustments can be made to future projects.
7. Replace and Retire
Don’t fall into the old IT trap of adopting new technology without retiring the predecessor. Once a data observability solution is implemented, all solutions that previously attempted to address these issues need to be EOL’d in order to expedite your time-to-value with getting benefits from data observability.
8. Have a Project Owner
Lastly, ensure that you have a project owner, who is tasked with responsibility to drive data observability outcomes internally, within the organization. This can bulldoze those final stumbling blocks out of the way.
Reducing Complexity Can Save Your Organization Money
Data complexity is an issue that won’t go away soon, and in fact, will only get worse. Data observability can help you address this problem to get more value from your data. It can also save your organization money.
In the case of PhonePe, Data observability saved them over $5 million dollars in annual software licensing costs while helping them scale their data infrastructure over 2,000%.
Learn more about data observability and how it helps enterprises reduce data complexity in Acceldata’s white paper – The Definitive Guide to Data Observability, view its 8 min product overview demo, or sign up for a custom product demo.