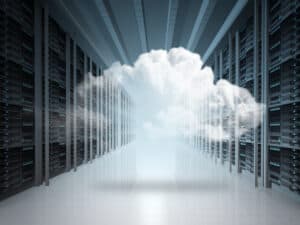
Businesses moving data into and out of cloud platforms often lack insights into data quality, utilization, costs, and more. Here, we interview Acceldata’s Tristan Spaulding where we discuss the need for and benefits of data observability in such environments.
As organizations move more and more data to the cloud and cloud data platforms, they often have an inability to monitor the environment. Specifically, they lack insights into performance, data quality, utilization, costs, and more. This can lead to degraded operations and high spend.
RTInsights recently sat down with Tristan Spaulding, Head of Product at Acceldata, to discuss these issues and how data observability can help. Here is a summary of our conversation.
RTInsights: What are some of the challenges in ensuring the reliability and optimization of data supply chains?
Spaulding: There are three challenges. The first challenge is just getting eyes on what’s out there. In many cases, there’s been a paved road that’s been paved over decades, of data going from warehouse table-to-table, warehouse-to-warehouse, and off to the iTools. One of the challenges now is that many of these pipelines are being developed in a more decentralized way by data engineers in code. So, the first challenge is just getting the eyes on all of this data that’s out there because some of the most critical elements might not be going down the paved road. That’s okay, but it does mean that some of the previous approaches may not quickly pick up. For example, airflow pipelines, they’re moving data.
The second challenge is scale. I mean that both in the scale of the number of data sets that people have, as well as the scale of the data moving through it. Both of those, of course, we know, have exploded. There are many more applications people have, and then the data is much larger. Understanding, and basically being able to apply and create with some level of automation, the rules and the checks that you need to have, is pretty significant. There are a lot of different approaches to how to solve this problem with pros and cons. Certainly, at Acceldata, one of the things we focus on is this scale and automation and using Spark to really make this effective.
See also: Multidimensional Data Observability
The third element I would call out is the increasing complexity and criticality of data reliability. What I mean by that is that, in the past, there were definitely some sanity checks that people were doing. Increasingly, as data pipelines are being used for externally facing applications, like machine learning models that are going to forecast demand or predict churn or provide a customized offer to a customer, these applications look for different things.
Machine learning models are sensitive to data drift in ways that dashboards aren’t necessary and may have more complex requirements. Being able to actually convey and enforce those more sophisticated or even more custom checks is another challenge.
To summarize, the big challenge is, first, catching the data because it’s coming from different sources. Second, being able to actually automate and apply rules and checks at scale, and third, seizing the richness of everything you need to capture from modern data pipelines.
RTInsights: Many aspects of day-to-day operations, in general, are impacted by difficulties finding skilled staff and keeping existing staff. Is that an issue here?
Spaulding: I think it is. There are two halves to it. The first half is if you look at the modern data, the so-called modern data stock, and all of the things in the perforate that as well, there are so many tools out there. There are so many options, and most of them are very, very good in particular ways. Determining which to use and when is the challenge. In many cases, the vendors will tell you, “Always use my tool for this.”
You could just take a couple of popular ones, like Snowflake and Databricks. These are incredibly rich platforms. Each is incredibly strong in particular ways, and they are updated all the time. Finding people that are able to understand when and how to use these tools, who are specialized enough in those tools and stay on top of them enough to wield them effectively, is really critical. They also need to be experts on any two, three, four, or five of these tools that you might have in your ecosystem.
That’s a really rare skill set and certainly something that at Acceldata, we definitely try to help provide some of that expertise automatically through our product.
The other side of this that becomes interesting is that there are companies that are cloud-native and have started with AWS, GCP, Azure, and things like that. They can scale as their companies grow. However, the majority of the largest companies in the world don’t have that luxury. They have decades and decades of investments in data platforms that are still running, that still need to run. Some of them might be migrated.
Some of them might be upgraded, but those still need to be operated. Keeping and growing the team that supports that while you’re also competing with these digital sorts of native challengers is quite difficult. Take the example of Hadoop. Finding people who are able to maintain Hadoop expertise and keep that system running while you’re getting a new or cloud-based system running stretches organizations. One of the things that we try to do is be aware of that, to be aware of the complexity. Don’t pretend everyone started in the cloud.
RTInsights: One thing that seems to help ease the labor shortage issue is to use automation to give the data engineers and others visibility into data pipelines throughout the data lifecycle. What is the impact on your data operations when you automate visibility?
Spaulding: The impact of automating data observability comes in two forms. The primary impact is around cost-effectiveness. These are sharp knives, and I would say in a good way when we talk about cloud data platforms. They do a lot really, really effectively.
On the other hand, mistakes can really burn you in a certain way. We’ve seen hundreds of thousands of dollars spent on a given query for one customer we work with. That wasn’t the platform doing anything wrong. It wasn’t any crazy thing the customer was doing wrong. It’s just that this particular setting was set up in a way, and a mistake was made, and it wasn’t caught quickly.
There’s an aspect here of applying checks automatically and having the expertise and the safeguards to protect against some of the worst runaway excesses that you would have. That’s one level of protection and optimization.
The second one is about making use of the best new features that these organizations release in the best way. Take Snowflake and Databricks, again, since these are the ones we focus on right now at Acceldata. These companies are releasing incredible features around performance and optimization all the time. It’s sometimes hard to, even if you’re an expert in this, retrain yourself every time on when to use query acceleration service, when to use this setting, when to use that setting, when is it more effective to do this, when to bump a warehouse up, or when to bump a warehouse down. It’s really complicated to do, even for a couple of workloads, let alone for hundreds or thousands of workloads that people might be running.
One thing data observability can help do is apply those actions automatically or give you a list of a suggestion. “Here are three things you could do. Here are the trade-offs. We think you should do it. Go ahead and apply.”
Now there’s a whole other half to this centered around the data itself flowing through. Here, again, the main impact is around cost. With the cloud data platforms, one of the big changes is that data reliability is not just a concern about eroding trust and sending bad data out to consumers, potentially to external consumers and customers as well. It’s actually a cost concern because all of these cloud data platforms offer pay-as-you-go pricing or burn-down credits-as-you-go. You run up costs and burn plenty of credits on queries on bad data, just as you do on queries on good data.
One of the opportunities that we see is to shift our data reliability as far left as possible toward the files, the applications, and the streaming systems that are moving data off of where it originates into more of the data processing data platform and data warehouse layers. By doing that, you not only improve trust and effectiveness, but you also avoid spending money on queries that don’t work so well. That’s one big side of what data observability gets you.
The other thing that it gets you is basically an increased ability to win with data. If you look at most organizations, they would like to make use of some of the emerging technologies out there, whether it’s machine learning technologies that can provide more accurate models or easier deployed models or whether it’s privacy technologies and marketplaces that allow you to actually monetize your data directly.
They would love to use these technologies to generate revenue. What’s missing is an understanding of data pipelines that exist, how effective they are, what they’re doing, and how they need to be fixed. We work with organizations that have hundreds of thousands of data pipelines. There’s a need for information that tells them which ones are being executed, when they’re breaking, and how much they’re costing. Data observability can really bring that level of understanding in and let them start to apply a real data strategy that leads to revenue generation from the data organization. I think that’s quite a new thing as well.
RTInsights: How does Acceldata’s multidimensional data observability help?
Spaulding: Acceldata is multidimensional data observability. It’s a long, long phrase, but the reason we say multidimensional is precisely that when you’re dealing with cloud data systems, you can’t separate the data from the data platform.
Take the classic example of data being late. You’re supposed to have this report updated. The data is late. Where’s the data? That’s a failure. The next question you would ask is, “Why is it late? And how do I prevent that from happening in the future?”
What Acceldata allows you to do, which is unique among anyone in the data observability space, is dig into the cause of such problems and fix them. For example, “Hey, this data is late because it’s using these queries. This warehouse that was processing these queries is now overloaded because it’s too small. Maybe you should increase that warehouse size, or you should add query acceleration service settings.” This is just taking a Snowflake example and how to improve that. That’s one direction of where actually diagnosing and fixing these problems faster is only possible when you’re able to connect the layers.
The customers we work with do end up with a list of pipelines, showing which ones are running and which ones are not. They are able to instrument these pipelines and get that view of what’s being executed, how good the data is going through, what it costs, and ultimately what and who it’s affecting downstream. They get a view of what’s working for the money that they spend on cloud data platforms and on these pipelines. They get to quantify the actual benefit they’re getting and how effectively they’re getting it.
If there’s a critical report or a critical application that is powered by a given Snowflake warehouse or Databricks application, people are willing to pay that cost. No one’s going to complain about that. If you’re sitting in the central group here, the central Snowflake group, you’re looking at the bill, you’re in FinOps, and you’re saying, “What’s going on with this? We’re spending so much.” It’d be great if you were able to line up that cost with the actual applications downstream. That’s not something you get without multidimensional data observability.
The two factors around the benefits we mentioned, around basically cost reduction or cost optimization and around winning with data, really only become possible when you’re able to connect the two key layers of the modern data stack. That’s what we call multidimensional data observability.
RTInsights: Can you give some examples of the benefits of using your solution?
Spaulding: The big benefits that we see are around cost reduction. There ends up being pretty consistently an immediate fix. It obviously varies by group, but there can be 10% to 20% workloads and queries that are simply not optimized or not effective at all. What I think is really powerful on this, though, is actually the rate of growth. What we do by aligning resources and applying optimizations automatically, and catching data before it breaks, means your rate of growth of spend on these cloud data platforms slows pretty significantly. It slows by about half.
Obviously, it’s going to vary by group as we go in. We can bend that cost curve. We’re able to do it pretty quickly because most organizations have built up a lot of usage on these platforms. The platforms aren’t going away. We just help them deliver value a bit faster.
Another benefit is on the winning with data side. One of our customers is one of the biggest data providers that are out there. They were able to get off of their legacy solution for the most part and basically apply richer data reliability experiences faster, cheaper, across more data because they’re able to use our engine versus the proprietary engine of their legacy vendors.
That helped them achieve the goal of, “Hey, we need to provide data for people. We need to know when data is wrong, and we need to push that back to the person that gave it to us. We need to know that for lots of data very quickly, without spending a ton on the infrastructure and the cost.” We were able to deliver improvements on all those dimensions.
Ready to maximize the return on your Snowflake investment with insight into performance, quality, cost, and much more. Visit Acceldata.io/snowflake