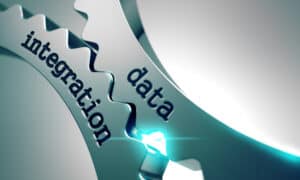
External data integration is critical in giving your organization the leading edge in making business decisions successfully.
As the demand for ESG disclosure and supply chain transparency accelerates, companies are faced with a new operational challenge: integrating external data. Many companies don’t know where to begin when it comes to calculating the potential cost of this data integration accurately. This often results in a cost underestimation of 35 to 70%. That range isn’t small, and you might be left wondering how these costs can be so vastly underestimated. Dataset maintenance is one of the most expensive at ~20% of the cost to build the pipeline, drastically underestimated due to the fact that the data quality from different sources varies significantly. However, it’s only one of six common underestimated costs your organization will encounter when integrating an external data feed into your current infrastructure.
The six critical underestimated costs of external data integration that are often underestimated can be classified as data acquisition costs or ingestion, operations, and maintenance costs. They are:
Acquisition Costs
- Data Scouting
- Data Trialing Standardization
Ingest, Operations, Maintenance Costs
- Data Onboarding
- Data Platform & Infrastructure
- Dataset Operations
- Dataset Maintenance
The benefits of integrating external data far outweigh the challenges that come with its implementation, but it is important to understand those challenges and how to address them. Once you know what to look for, identifying and estimating these underestimated costs can make you an external data expert, ready to successfully navigate the world of third-party data and reap the benefits.
See also: Poor Integration Hinders Digital Transformation Success
Data Scouting
The costs here can be internal or external, whether it’s an in-house role dedicated to searching for data sources or a third-party team. While this makes sense as the obvious first step and has clear price tags tied to it, what’s overlooked is where the cost sits and who’s footing the bill. In large organizations, it isn’t uncommon to scout the same data set for more than one team. But that means paying for it more than once as well, and that cost can quickly add up across departments. Another mistake often made here when estimating costs is only considering the cost of licensing a data source once it’s identified, without consideration of the cost of the resources used to allocate it. As data scouting is the first step in external data integration, it’s critical that these costs are taken into account.
Data Trialing
Estimating the costs of data trialing can be a bit tricky, as there is no way to know ahead of time what the state of the dataset is, or if it will be utilized for your organization’s needs post-trial. Costs associated with data trialing often stack up when you consider the time and effort your data operations team must put into setting up and then evaluating the dataset as a source. Trialing can quickly become a sunken cost, but it’s relatively easy to estimate. Use the data engineer(s) hourly rate and multiply it by the number of hours needed for cleaning, wrangling, and testing the data against the use case. It’s estimated that data trialing and evaluation is a 15% cost over time.
Data Onboarding
Once a dataset has been identified, there are a series of questions to ask to help calculate the costs of building a data pipeline to onboard the data. This part of the implementation process includes quality checks, schema changes, and other transformations to the data to make it usable within the current infrastructure. So how do you determine the cost here?
First, ask:
- What format is the data in?
- How large and complex is this dataset?
- How many data frames does it have?
- How much historical data is available? How much of it do I need?
- How much of the overall dataset needs to be loaded? 100%? 50%?
- What other responsibilities does my data engineer have for the next month or more that need to be offloaded to another resource?
As a baseline, assume the average data engineer can onboard between two and four datasets per month IF that is their sole responsibility. Once these questions have been answered, the number of hours required to onboard the dataset(s) can be estimated. Then multiply that number by the data engineer’s hourly salary to get the cost of this stage. The costs can really stack up here when assumptions are made about the bandwidth of your data engineer(s). It’s critical to understand the full scope of work that’s involved in onboarding and ingesting a third-party dataset, to properly allocate resources. Overloading engineers can cause burnout and attrition rates to increase over time, so understanding the full scope of work for external data onboarding and ingestion is important for determining the estimated 10%+ cost overtime for this work. Without properly calculating the costs, the financial impact increases as new datasets are onboarded.
Data Platform
This is not just a question of building versus buying a data platform but rather a summation of costs for building (or buying), including associated costs for ongoing maintenance, resources, and time-to-value. The upfront cost of building a custom solution may be appealing, but it often excludes the cost of maintaining and updating the platform as data, needs, and strategies change. It’s important to consider who is responsible for this effort–is it someone on the internal team, or is it an external service provider? Regardless of who it is, knowing the costs associated with both can help provide a clearer picture of the total cost of the platform instead of just focusing on the cost of its acquisition.
Dataset Operations
Without a doubt, dataset operations is the most overlooked aspect–and thus the most underestimated–cost associated with external data usage. Datasets are often thought of as static things, but the reality is that they are not. They require ongoing monitoring and attention that is often missing when creating a budget for an external data pipeline. These tasks increase in severity if the data pipeline is mission-critical, which requires data operations teams that work 24/7. Operational tasks include vendor delivery and outreach, updates, feed updates, and delivery and quality verification to start. Operational costs also include those needed to adjust, update, or maintain your infrastructure as well–this includes the technology and the employee costs associated with this work, just as it does for data operations engineering. A conservative estimate for these costs is calculating 10% of the initial build cost per year and then adjusting as needed.
Dataset Maintenance
As we just established, third-party datasets are not static things. They’re a living entity that provides exponential value IF their integrity is maintained. This part of implementation is often forgotten when it comes to external datasets. But forgetting maintenance needs and costs can quickly deteriorate the value each dataset brings to your organization. Common dataset maintenance tasks include field and schema changes, introducing new data frames, merging historical data, and bug fixes. Most data suppliers ease these costs with change notifications but may come at an additional cost. If a dataset isn’t properly maintained, the results can be catastrophic for mission-critical data that is silently undermining the accuracy of your business. Maintenance costs may also increase with scale and require more attention for more external data. Maintenance costs need to be calculated over a multi-year period that accounts for growth. Approximately 20% of build costs should be allocated to maintenance.
A last word on data integration from external sources
In summation, your data engineers work hard, and the best way to support them in delivering a quality external data pipeline is by understanding the depth and breadth of the work that goes into its implementation. External data is critical in giving your organization the leading edge in making business decisions successfully, and setting up your data foundation well is the best way to do that. They say the early bird gets the worm, but the second mouse gets the cheese–suffice to say, those who are prepared win in the end, and that rings true for external data integration.