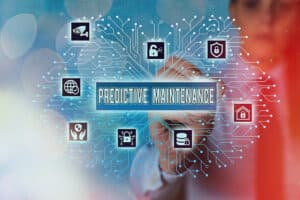
Using analytics and AI, OEMs of connected equipment can gain a deep understanding of the status of a machine and move to a predictive maintenance mode of operation.
Maintaining consumer and industrial equipment in homes, offices, plants, on the road, or in the field is an expensive proposition. Sending maintenance staff on frequent trips drives up operational costs and does not scale well. The long-standing issue is becoming more problematic as OEMs move to equipment-as-a-service models where they are responsible for maintaining the equipment throughout its life span. As such, many OEMs are looking to leverage the connectivity of modern equipment to institute predictive maintenance practices.
One of the greatest benefits of connected products is the wealth of data available about their operation and health. Companies can use that data about the product (and combine other data such as its location, operating environment, etc.) and gain great insights into its performance while operating. Analysis of this data can help identify problems in the making or spot analogies that require additional attention.
Such remote monitoring capabilities can be used in several ways. For example, rather than sending a technician to every site simply to check the health of a piece of equipment, that basic information can be viewed and collected from a central location. Connected equipment makes that possible. The embedded connectivity provides a conduit to share equipment status and health data.
An additional benefit of having a connected product and using remote monitoring is that once insights are derived about the state of the equipment, a company may be able to make changes (e.g., reset the product or update its software) without actually visiting the site.
See also: How Will Prediction Models Impact the Future of Vehicle Maintenance?
The road to predictive maintenance
Equipment failure or degraded performance can have significant negative business implications, including lost revenues, customer dissatisfaction, and damage to a company’s reputation.
To avoid these problems and improve operations, OEMs are moving away from traditional maintenance methods where a company waits for something to break and then fixes it. Reactive approaches like this result in disruptions and unplanned downtime.
A better approach is preventive maintenance, which uses manufacturer data on the average lifetime of equipment or its parts along with historical data on an item’s repairs and breakdowns. With such information at hand, OEMs can schedule maintenance before a failure. If a part typically fails in four years, the maintenance team replaces it in, for example, three years and eleven months.
The benefit of this approach is that unscheduled downtime and maintenance is avoided. Additionally, by planning maintenance in advance, OEMs can ensure required parts are in-house, and they can allocate staff time appropriately.
The limitation of this approach is that it relies on the law of averages. All assets of the same type are created equally. That is troublesome in two ways. First, equipment might fail before its average expected lifetime. That leaves the user in the lurch, and the OEM must rapidly dispatch someone to the scene to fix or replace the equipment. Second, good equipment may be replaced sooner than it needs to be just to avoid problems down the line. While that avoids the negative impact of a failure or downtime, the OEM does not get full value out of the equipment.
A better approach, which is rapidly gaining favor, is proactive maintenance. This method complements the vendor and historical information with real-time data about the current state of a part, device, machine, or piece of equipment. Such information can be used to avert problems. For example, based on past experiences, an OEM might know that a particular piece of equipment is likely to fail within a day if it starts running hotter than normal. Armed with this knowledge, an OEM could immediately repair or replace the item anytime that equipment’s temperature spikes.
Predictive maintenance is the next step up in the level of sophistication for maintenance. It typically is defined as maintenance that uses analytics and artificial intelligence to spot trends that might lead to equipment failure.
Specifically, predictive maintenance is based on analysis that uses real-time and historical data about an asset to spot trends that might lead to failure. It spots changes and can make inferences about the failure of an asset. Such capabilities can help maintenance staff identify parts or equipment that are likely to fail before their scheduled replacement and maintenance time. When such an expert system detects a problem, it can trigger corrective action based on information derived from collective years of knowledge about a piece of equipment.
See also: Out of the Frying Pan and into the Connected Kitchen
Connectivity is a game-changer
Connected products can deliver a wealth of data and provide real-time insights into the operating state of equipment in the field. OEMs can leverage connectivity to remotely monitor equipment and make some repairs and updates, such as installing new software or firmware.
Additionally, when analytics or AI is applied to the data, an OEM can gain a deep understanding of performance levels and move to a predictive maintenance mode of operation.