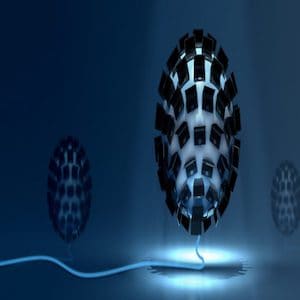
Moving toward next chapter: Training AI models closer to where data is collected at the network edge.
SAS Institute is betting that one of the primary use cases of artificial intelligence (AI) will soon revolve around applying predictive analytics to processes running at the network edge in real-time.
The company is investing more than $1 billion, in part, to enable AI models to be trained where data is being collected at the edge, says Oliver Schabenberger, executive vice president, chief operating officer, and chief technology officer for SAS. The goal is to advance AI use cases involving edge computing scenarios, he says.
Cutting Down on Cloud Data Transfer
That will be a big change. AI today requires moving massive amounts of data into a single location where machine and deep learning algorithms can be cost-effectively applied to training a model. That AI model is then applied to with the context of an inference engine.
Right now, much training of AI models occurs in the cloud using graphical processor units (GPUs), which have proven more efficient at processing machine and deep learning models. Traditional x86 processors, and sometimes GPUs or other emerging technologies, are employed. These run the AI inference engine created by AI models – ideally as close as possible to where the application augmented by the AI model is running.
See also: SAS Opens Wallet Wide with AI Investment
The big problem is deploying and dynamically updating AI inference engines against ever-changing real-time data. Some use cases involving streams of real-time data with little variance are fairly simple to augment. It’s done by using an AI model that has been trained to recognize that data.
But in use cases involving an automobile, for example, new types of data are constantly being streamed into the environment. That makes it tough to predict every possible scenario. So while a red light is highly predictable, a child chasing a ball onto a rainy road is not.
Fortunately, as compute horsepower available at the edge becomes increasingly affordable, it will become less necessary to move massive volumes of data into the cloud to train an AI model, explains Schabenberger. “It will be possible,” he says, ” to train AI models at the edge.”
Build or SaaS?
SAS has already created the SAS Analytics Center of Excellence to help organizations improve AI skills by certifying professionals in AI and Machine Learning.
But even well-trained organizations will need to wrestle a key question: Should they consume an AI model as a service? Or build one from scratch? Schabenberger says many AI models being created to augment a specific process will be consumable using software-as-service (SaaS) delivery models. So it might not make much economic sense for organizations to duplicate readily available AI models which are continuously trained by experts in the area, he says.
Whatever the AI path, SAS believes the AI genie is already unbottled. Organizations, he observes, are investing in AI out of both fear and enlightenment. Business and IT leaders fear a competitor will apply AI to a business process, rendering rivals obsolete overnight.
Right now, however, most AI use cases are relatively narrow. Today’s AI today can be relied on to augment human reasoning or automate tasks with a predictable set of narrowly defined events.
Soon enough, though, AI models applied in real time on edge computing platforms will take advantage of 5G networking services to train AI models in real time. Once that begins to happen, the next phase of AI will finally begin to catch up to imagination.