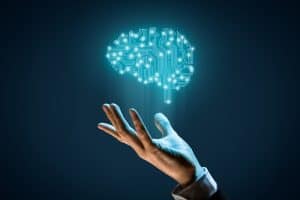
Edge computing changes are enabling decision-making where data is generated, and are powering applications that are reliable, private, and faster than ever.
Edge computing still isn’t the go-to framework for processing data, but more companies are making the shift. After a decade of centralizing cloud processing, more organizations will look to edge computing to reduce latency and scale processing. Here are some factors influencing this shift.
Before neural networks changed how we process big data, cloud computing sped up processing by bypassing on-premises solutions. Businesses sent data to the cloud for processing and received insights back with barely perceptible latency.
See also: Edge Computing Enhances In-Store Retail
Now, with more IoT devices, more data, and more systems, latency is perceptible. Artificial intelligence and machine learning allow companies to run their algorithms much closer to the device.
Edge computing helps balance two major needs:
- Data appetite: Our data production is incomprehensibly big. Companies have to move this data in real-time to produce competitive insight, creating enormous bandwidth needs, storage needs, and heavy computing costs, solved by centralized cloud computing.
- Privacy: This data movement to a centralized cloud creates privacy issues for sensitive data. Companies previously solved this by adopting a safer but significantly slower, on-premises processing.
AI/ML-optimized solutions allow companies to move processing more efficiently to the edge. They help protect sensitive information but avoid high computing costs by leaving that processing distributed at the edge. McKinsey predicts that AI will generate an extra $13 trillion of economic activity by 2030, and some of this will be in edge computing.

Containerized applications are more common
Containers are standardized, standalone software solutions designed to work independently. It includes everything you need to process a certain task or task series. Because they isolate software from the larger environment, you can export this process to other locations without risking corruption or misalignment.
Edge containers provide:
- Low latency: Instead of far-off cloud processing, edge containers remain close to the user.
- Scalability: Edge containers are deployed in parallel to points of presence (PoPs), so organizations can send them to many points at once. Processing grows as companies expand, and companies can meet demand where they are.
- Lower bandwidth: Data-hungry applications cost companies a lot of money because traffic to a centralized server is high. Edge containers break up this traffic and provide pre-processing to reduce the load.
- Maturity: Containers are tested and deployed as all-in-one solutions. There’s no need for retraining, and developers don’t worry about misalignment due to the processing location conditions.
See Also: Center for Edge Computing and 5G
Lean edge computing solutions
TensorFlow Lite and TinyML are just the beginning. They provide deep learning frameworks — traditionally deployed on processor-heavy applications — for on-device inference. They’re extremely low power and build sensor analysis into even the most extreme edge applications.
The key takeaway is flexibility. Companies can deploy low bandwidth, low latency solutions that previously required computationally intense (and expensive) commitments. Edge computing is becoming more common because it offers organizations more choices about how, where, and when to deploy. The introduction of new tools like edge containers and TensorFlow Lite offer deep learning capability without the weight.
Edge computing is now possible in so many use cases we previously never dreamed of. And thanks to decision-making close to the device, these applications are reliable, private, and faster than ever.