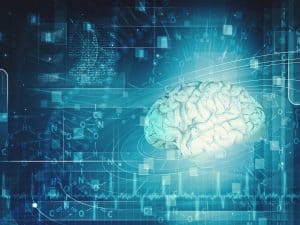
Today, there is a need to transition from simply analyzing streaming data to developing insights that understand events in real-time.
Data insights have an expiration date and can spoil. Take an extreme but simple example. An autonomous car using AI-based video analysis to avoid accidents by spotting objects in the road cannot act an hour after a broken-down car in the same lane is detected.
Similarly, waiting a week to act on a predictive maintenance system’s recommendation to replace a potentially bad part will likely result in downtime.
Spoiled insights work the other way, too. Repeatedly analyzing data generated once a day, every second will not return and new insights based on the current state of a device. Furthermore, taking action in seconds on stagnant data may miss a long-term effect.
How do you ensure your data insights are good to use (ripe) but not too young (not ready) or old (spoiled)?
One way to approach the issue is to match or assign the type of insights with an expiration date. In work done about a year ago, Forrester suggested there are four classifications that can help. They include:
- Real-time insights, which are valid for sub-seconds or seconds and are typically acted upon by automated systems. Example: cart-based recommendations online.
- Operational insights, which are valid for seconds to hours and usually relate to business processes. Example: delivery schedule optimization for shipments.
- Performance insights, which are valid for hours or weeks and usually relate to key performance indicators. Example: understanding and addressing product quality issues.
- Strategic insights, which are valid for weeks, months, or years and usually relate to executive-level decisions. Example: developing a new product line based on market opportunities.
Such an approach can have great implications on how analytics and data management solutions are architected. And each classification has different data and insights expiration times.
For example, data for real-time insights can quickly go out of date because it is generated rapidly, then changes, then expire. The suitable analysis solution must be able to capture this data and make use of the derived insights to ensure old recommendations don’t result in useless, or worse yet, harmful decisions.
Strategic insights face an opposite, but similarly troubling, problem. Without fully understanding the longevity of the strategic insights, some organizations are discarding them too early. In doing so, they miss out on the value that those insights could have provided for some of the company’s most important decisions.
Use before the expiration date
Keeping insights fresh and relevant requires new ways of thinking about analytics processes.
Is data being analyzed too slowly, the reports of derived insights being delivered too slowly, or has the data changed before a decision or action can be made? If that is the case, the information is of less value for operational purposes.
If insights to often are outdated before they are used, accelerating the data-to-insights-to-action process is crucial. Businesses must examine the end-to-end analytics operation to determine where breakdowns occur that cause problems. If data being analyzed too slowly, it may be an indication that the analytics technology should be improved. On the other hand, if reports are being delivered too slowly, it might require re-evaluating business processes or reprioritizing a more timely distribution of insights once they are ready.
A move to Continuous Intelligence and event processing
A recent blog about Big Data and analytics noted: “It’s no surprise: most companies working with stream data today say they are planning to make changes to drive greater value. Advancements in machine learning (ML) and very-high-speed data persistence for real-time analytics are reshaping strategies and architectures. In addition, 88 percent of surveyed companies say they need to perform analytics in near-real-time on stored, streamed data.”
Today, there is a need to transition from simply analyzing streaming data to developing insights that understand events in real-time. When businesses have such analytics capabilities, they can use that information to decide plans of action. Such capabilities are essential for both real-time and operational insights.
Enter Continuous intelligence (CI). CI gives organizations the information needed to react to events as they are happening. It is a form of real-time analytics used to support decisions when actions must be taken in milliseconds to minutes.
CI learns from real-time and historical data using artificial intelligence to gain insights into events as they are happening. CI goes beyond streaming analytics, which generally only applies a few filters, transformations, or aggregations to data. Like the nervous system, CI propels people to act immediately.
To work, CI relies on platforms, architectures, and software that allows organizations to collect, organize, and analyze data, enabling fast actions in response to real-time events. The data can include email messages, clickstreams, social media, data logs, and information from sensors and the Internet of Things (IoT) devices.
Another differentiation between CI and analytics of real-time data is that CI enables event processing by analyzing streams of information about things that are happening now. In other words, CI is a method for processing real-time events and extracting information from event streams as they arrive. The goal of such event processing is to identify meaningful events (such as business opportunities or threats) in real-time situations and respond to them as quickly as possible.
In most cases, CI makes use of artificial intelligence (AI) and machine learning (ML) to complement traditional analytics used to investigate event data. Businesses can realize significant benefits from CI when their real-time analytics capabilities are integrated into their operations. In this way, CI can prescribe actions in response to business moments and other events.
Using CI on the fastest generated data will deliver timely insights that can be used to make decisions in the moment. If the intelligence is embedded into business processes so that actions are automatically taken, the instantaneous nature of the end-to-end process (data ingestion-analysis-action) means insights are applied as they are created and thus do not expire.