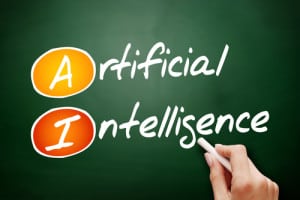
Businesses are showing more maturity when it comes to AI benefits and potential achievements.
Successful development and deployment of artificial intelligence rely on a defined, deliverable plan of action, open from the beginning to multiple sectors of a company.
Forrester Research suggests that machine learning operations (MLOps) is the solution to achieving that. MLOps is the best way to ensure an AI development cycle sticks to its original goals while keeping it well supported in the future, principal analyst Mike Gualtieri noted in a webinar.
One of the first, and most difficult, steps is finding a use case. As a Gartner webinar mentioned, 26 percent of business leaders surveyed said finding a use case was one of the three most difficult AI challenges. Understanding the benefits of AI, once deployed, is equally as important, to avoid unsatisfactory end results.
Businesses are, on a whole, showing more maturity when it comes to AI benefits and potential achievements. In another Forrester study, Gualtieri said organizations are aiming for initiatives that are more in line with a mature market, such as customer experience, profitability, and revenue improvements.
“Customer experience, profitability, and revenue growth are always in style, but three years ago those goals would not be at the top,” said Gualtieri in a webinar related to the study. “AI was often associated with new products, services, and research and development. While these use cases are totally valid, they’re much harder to invest in because they’re new.”
Next Steps
Once a use case is found and goals between different sectors are defined, the next step is ensuring it supports multiple formats, dependencies, and open-source models and infrastructure.
“We have to get used to machine learning infrastructure being in the cloud and an ever-evolving set of proprietary and open-source components as the new normal,” said Gartner director analyst, Peter Krensky, in a webinar. “Every year there’s a new open-source framework that we weren’t talking about two years ago and now we’re talking about all the time.”
After deployment, development isn’t finished. In Krensky’s webinar, he showed the operationalization cycles, in which the AI model is continuously updated and improved upon. This can add greater value over time, and not require as many resources as building a new model from scratch.
Learn about additional steps and Dell’s approach below or view the PDF here.