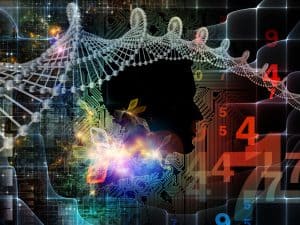
Operationalizing AI depends on a mature infrastructure, highly skilled staff, and elimination of data silos. When these are achieved, companies can personalize customer experiences at scale, boost profits, and gain a competitive edge.
Between the recent government announcement that data access is at the center of new AI initiatives and the ongoing conversation around how to mitigate bias in AI, there is one fact that tech leaders can agree on: artificial intelligence technologies are no longer just a “nice-to-have” — they are a core capability for enterprise-wide digital transformations. And while operationalizing AI effectively can seem daunting, the resulting ability to deliver a better customer experience and gaining (or retaining) a competitive edge in the market prove that the rewards far outweigh any risks. However, common myths about operationalizing AI prevent enterprises from even embarking on a journey to implement AI solutions.
A research report published earlier this year shows that companies believe operationalizing AI can create quantifiable value and provide insights about their market, customers, and competitors to improve business outcomes. However, the research also reveals that leaders feel they are limited by operational silos and struggling with data strategy challenges and a lack of resources.
But before we dive into solutions for these challenges, what does it mean to operationalize AI? At the core, it’s all about integrating AI into platforms and systems to elevate business outcomes. AI is successful when enterprises are able to respond with speed and scale to data insights. As one leader interviewed for the report said, “Insights are worth a dime. Actions are worth a dollar. Operationalizing AI allows us to drive system behavior based on insights coming out of our AI model.”
So, what do you need to know to effectively operationalize AI?
Proving the efficacy of digital decisions is essential but daunting.
Decision-makers understand that defending and proving the efficacy of their digital decisions is important, but 58% report it’s extremely challenging to do so. Why? It’s because machine learning models (ML) are unreliable without use case-relevant context, ongoing model updates, and transparency into the factors that affected a prediction. To truly scale AI, it’s imperative that organizations have comprehensive digital-decisioning capabilities that incorporate decision modeling, ML, human decision logic, and other decisioning technologies and techniques.
As impactful as AI sounds, the outcomes are only as good as the data inputs themselves. If biased data is incorporated into the training of the machine learning model, you could see biased predictions and recommendations, which can lead to costly fines, negative customer experiences, or loss of market share.
The risk of biased outcomes points to the need for insights from analytics and real-time data and model updates as part of an ongoing feedback loop. Additionally, the potential for biased predictions and recommendations envokes the need for explainable AI solutions that provide transparency into ML predictions and the factors driving the outcomes. As the speed of business increases, it’s essential to be able to analyze, in real-time, the weighted factors driving the prediction.
Collaboration and data challenges slow success
AI decision-makers are keenly aware of the roadblocks on the path to AI project success. According to the research, data is the top reason for a lag in organizational adoption of AI. More than half (51%) of decision-makers say their organizations have too much data to make collaboration efficient, and 42% of decision-makers struggle to identify — and gain access to — the right data. What’s the solution? Break down organizational silos that hinder accessibility to data and prevent collaboration between subject matter experts and data scientists.
It may seem challenging to hire good data scientists right now; many in the industry are feeling the pinch of qualified tech labor. However, finding someone with a Ph.D. in statistics is not the only way to begin operationalizing AI; businesses should consider candidates with unique backgrounds and augment their teams with consultants who can provide expert guidance. Additionally, companies should create clear communication channels and facilitate collaborative feedback by prioritizing strong communication between departments and eliminating silos. The report cites departmental silos as one of the top three collaboration challenges firms face; the fact that one in four organizations don’t embrace a culture that encourages data democratization makes matters worse.
To keep the momentum of AI projects, it’s crucial to ensure data scientists and their teams have a direct line of communication with CTOs and executive leadership. A best practice for ensuring the incorporation of real-time data into business initiatives and planning is to hold regular meetings with leadership and stakeholders. These meetings ensure consensus on how to leverage data and automation to improve bottom-line initiatives such as customer service, competitive market positioning, or operational efficiencies.
AI use cases are prevalent across different business functions
At least one-third of decision-makers report that they have too many use cases for AI projects across sales, marketing, and customer experience. For example, fifty-three percent of CX leaders reported that they have too many AI use cases/application scenarios to manage. Conversely, leaders in finance (45%) have identified a “manageable” number of AI use cases/application scenarios.
The top use cases for AI projects are insights-driven as leaders want to understand their competitors, the market, and their customers. Additional priorities for exploration include security, innovation, and automation. For example, the financial services industry is turning toward automation to translate handwritten documents to actionable insights more quickly and accurately than humans.
A look toward the future
Forrester’s research reveals that 68% of enterprises are implementing AI, and the firm forecasts that nearly 100% of enterprises will implement some form of AI by 2025. Industry leaders’ confidence in their organizations’ ability to successfully operationalize AI ultimately depends on a mature infrastructure, highly skilled staff with a commitment to collaboration, and elimination of data silos. When these are achieved, companies can personalize customer experiences at scale, boost profits, and gain a competitive edge.