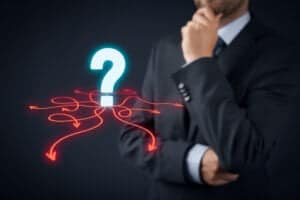
Decision intelligence leverages AI-driven automation and data analysis to uncover key drivers, patterns, anomalies, and segments that are not easily visible.
Businesses today have massive amounts of data and increasingly complex business decisions to solve with this data, but getting fast analytics and insights is not a simple task. In fact, a survey from NewVantage Partners says that just 24% of firms identify as data-driven, and Gartner found only 30% of employees use analytics and business intelligence (BI) tools. Not only are there few numbers of employees using these tools, but they might not even have the proper tools at their disposal. The problem with traditional business intelligence tools is the focus is on reports, visual dashboards, and other forms of descriptive analytics. Modern data organizations leverage a range of descriptive, diagnostic, predictive, and prescriptive analytics to inform insight-driven actions but suffer from siloed approaches using disparate tools, which slows down the overall analytics workflow. Enter decision intelligence.
See also: The 4th Shift in the Evolution of Finding Insights from Data
Decision Intelligence offers a way to bridge these insight gaps, helping users make better, faster, insights-driven decisions at scale — with continuous and evolving improvements. Decision Intelligence has rocketed from obscurity to a Top Strategic Technology Trend for 2022 by Gartner, who predicts it will be practiced by 33% of large organizations by 2023. But how can organizations determine which solution they need, decision intelligence or BI? Here are three ways decision intelligence differs from business intelligence.
1) Decision intelligence bridges BI and Data Science (i.e., Descriptive, Diagnostic, and Predictive Analytics)
Teams are becoming bootstrapped, working with fewer data science resources and making dealing with multiple data requests a day, and completing faster, deeper analysis a challenge. BI is suited for descriptive analytics to answer which KPI or metric changed. Although users can easily access the what, they can’t always obtain the deeper content of the data they need to support their action and decision-making — the why — when using legacy BI tools. Thus, the analytics consumers must rely on analytics creators, which slows down decision-making.
Decision intelligence expedites identifying the what (descriptive analytics) underlying in the why (diagnostic analytics), making the how (predictive and prescriptive analytics) within data easier to find. To put it simply, predictive analysis is what will happen, and prescriptive is how it will impact outcomes. Access to this information drastically reduces the time and effort necessary to make strategic, tactical, and operational decisions. This is critical when building a data decision-driven strategy.
2) Powerful for analysts and easy for business teams
BI is focused on robust reporting and reporting functionality but lacks the more advanced analytics capabilities, automation, and powerful functionality expected by data experts and analysts. On top of this, organizations either cannot hire enough data science talent or are underserved by their existing team, making it more difficult for businesses to gather critical data insights. Job listings for data science and analytics reached around 2.7 million in 2020, according to TechJury, meaning it is hard for companies to recruit and hire expert analysis and data scientists who can work with their data. Organizations need tools that enable analysts to gain the advanced abilities of data scientists and produce the analytical insights the business needs.
Decision intelligence, on the other hand, is suited for both analytics consumers and analysts. Decision Intelligence greatly simplifies the complexity of decision-making by automatically translating business questions (using natural language) into big-data search queries. Analysts and data experts can automate data analysis and generate the types of insights that may traditionally be reserved for data scientists while also allowing them to use tools familiar to them, such as SQL and Python. Regardless of data skills, decision intelligence allows these groups to flexibly explore and analyze data in plain English, democratizing exploration and analysis by making it as simple and intuitive as a Google search.
3) Decision intelligence includes intelligent automation
BI focuses on visualizing aggregated data instead of discovering granular insights from volumes of unaggregated data. Decision intelligence leverages AI-driven automation and data analysis to uncover key drivers, patterns, anomalies, and segments that are not easily visible. Without intelligent automation, analysts, data scientists, and IT teams are forced to crunch data, which can take hours or even days manually. Because of this manual process, oftentimes, teams miss important insights.
According to the Smartsheet Report Automation in the Workplace, nearly 60% of workers surveyed estimate they could save six or more hours a week — almost a full workday — if the repetitive aspects of their jobs were automated. Decision intelligence is defined by capabilities like automated visualizations, automated insights, automated prep, autoML, and proactive intelligence. This not only democratizes data analysis tools so anyone can discover insights, but it also automates the process to make it more efficient and reduces the chance of risks related to manual errors.
Because there are many analytics tools on the market, it can be difficult to discern which one can actually help organizations become more data-driven. Any organization that is impacted by the current talent shortage, and is wasting talent on manual data analysis, should consider the key differences between DI and BI. With the ability to put AI-powered analytics in the hands of the everyday user, accelerate analysis and automate manual tasks, decision intelligence is making it easier than ever for organizations to become data-driven.