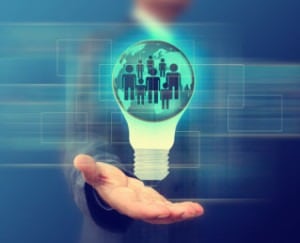
Companies should consider a multi-faceted approach to recruited artificial intelligence (AI) and machine language (ML) talent.
The race to hire artificial intelligence and machine language talent is more competitive than ever. Enterprise executives are demanding greater automation in all sectors of business and that means hiring and retraining.
Is there a sweeping answer to the AI talent challenge? No, but there seem to be plenty of narrow fixes. Together they can help organizations acquire or develop people who will develop and run AI and ML initiatives.
Fix Number One: Don’t try to copy the enterprise-wide AI strategies used by tech giants such as Google and Facebook. Those companies were built on big data, vast collections of diverse data types that formed the core of the businesses.
Rather, the average company looking at AI and ML is likely to focus on a well-defined need. Think of applications tied to use cases such as consumer fraud detection and product quality assurance. Recent thought leadership examples highlight how use of AI and ML in that sort of narrow application might be manageable for many organizations.
What experts say
Andrew Ng, writing in the Harvard Business Review, outlined a focused view for AI and ML. “Sure, it has transformed consumer internet companies such as Google, Baidu, and Amazon — all massive and data-rich with hundreds of millions of users. But for projections that AI will create $13 trillion of value a year to come true, industries such as manufacturing, agriculture, and healthcare still need to find ways to make this technology work for them. Here’s the problem: The playbook that these consumer internet companies use to build their AI systems — where a single one-size-fits-all AI system can serve massive numbers of users — won’t work for these other industries,” he wrote.
“Instead, these legacy industries will need a large number of bespoke solutions that are adapted to their many diverse use cases.”
A company like Facebook may utilize many thousands, even millions, of data points to train an AI or ML solution. However, a manufacturer looking to use ML and AI to detect flaws in a product might have only 50 data points, according to Ng. Yet, what Ng and other experts note is that the manufacturer might have dozens of experienced line workers who already know what flaws to look for in a product inspection. Those staff members can help to train the ML app by sharing their knowledge.
In fact, many niche AI and ML initiatives can benefit from such knowledge sharing. That and other techniques that add a business perspective to the project.
Who builds the app?
The manufacturer can’t compete with the $500,000 salary that Google might pay a job candidate with a PhD and deep knowledge of AI. However, it does need to hire someone with AI and ML tech skills to lead the AI team. In addition, software engineers are needed to build out an application. Those engineers may be hired from the IT team or outside sources. However, that analytics talent business leaders who understand how the company operates can supplement that tech talent.
Those business unit leaders may need to learn how AI can or can’t be used, which is an issue being addressed by companies such as Levi Strauss & Co. At Levi, some business leaders are going through a crash course in advanced analytics and data-based applications.
Levi describes its initiative as a two-stage strategy. Stage one was to shore up its tech talent. The second stage is to ensure that others in the company “have the data literacy to connect with these data scientists, coders and others, or prime them to fan out and bring innovation and efficiency to every corner of the company”. Those business leaders may not turn into developers, but they can learn how to put AI to work.
Team construction
In a recent article, Gramener co-founder Ganes Kaseri identified four keys to hiring and developing an AI and ML team.
One approach he described was to maintain a “self-identified list of skills for every employee”. Then they should update that list every six months as employees. That enables leaders to look beyond the existing IT team for employees with less obvious technical skills.
In addition, he suggested that organizations tailor their data science curriculum using public content from the web. He said formal training programs often fall short of an individual organization’s needs. He also echoed the calls to include domain expertise on the AI and ML team. Finally, he encouraged companies to “enable experimentation and learning on the job. “The best courses and training methodologies will amount to nothing if you don’t let your teams experiment, make mistakes, and learn on the job”.
Developing artificial intelligence and machine learning talent in house shapes up as a smart way to fill out an AI or ML team. Organizations still need team members with specific AI and ML technical skillsets, such as AI developers and AI engineers. Together they fill out an effective team.
The price for expertise
Yes, an organization is going to pay a premium for that type of technical talent, although it doesn’t have to be in the $300,000 to $500,000 range where the tech giants recruit. Take the example of an AI engineer who is going to design and build an application. The average US salary for an AI engineer, according to ZipRecuiter, is $164,769. In Washington state, home of Microsoft and other tech companies that average jumps to more than $190,000. But companies in North Carolina are paying only $132,000 on average.
Remember, that pattern reflects the way tech salaries have trended for decades. The highly competitive/high cost of living markets like New York, California, Seattle, and Washington, D.C., usually pay better. Companies in smaller markets still must sell any tech job candidates on factors such as local quality of life, company stability, non-cash benefits and telecommuting.
Even when companies must pay a premium for AI talent they can justify the expense by the bottom line return if they target the right use cases. The product inspection application mentioned may keep a valuable customer happy and returning for more business. Does that translate into new revenue? Can another company justify higher AI salaries if AI-based apps justify themselves by cutting back on billing fraud or reducing security breaches? It’s worth an analysis.
The rush to artificial intelligence and machine learning adoption isn’t driven by what some experts thought a few years ago: Driverless cars and virtual presence robots. The next wave of AI projects shapes up as seemingly mundane line-of-business applications. Yet, chosen well, they will address carefully defined needs and offer direct revenue/cost improvements on the bottom line.