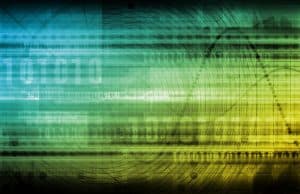
Advanced graph analytics uses real-time information to figure next best actions to prevent fraud, increase product sales, offer critical healthcare patient support, and more.
We are swimming in a sea of data. It’s been estimated that more 2.5 quintillion bytes of data are created every single day – and this number is continuing to increase. Every industry – from healthcare and banking to eCommerce and retail – requires the processing of large amounts of data in seconds. Traditionally, these industries relied on relational databases to store, process, and retrieve data. But modern, real-time data demands have rendered relational databases obsolete. Today’s organizations require advanced graph analytics that can both process large amounts of data and offer meaningful and actionable insights.
See also: How the World’s Largest Banks Use Advanced Graph Analytics to Fight Fraud
Graph analytics allows you to drill down into complex interrelationships among organizations, people, and transactions. In fact, Gartner analysts have highlighted Graph Database & Analytics as a “top 10 trend for data and analytics,” with an estimated annual growth of 100 percent annually through 2022. The ability to offer a “next best action” for a customer or organization is what separates regular analytics from next-generation advanced graph analytics – and these specific insights can mean the difference between a sale or a loss, or even life and death.
Advanced Graph Analytics and the “Next Best Action”
There are three must-have capabilities for real-time advanced analytics “on the graph,” or “advanced analytics on connected data sets for the next best action.” The first is a transactional or mutable data infrastructure. In the case of a payment, the advanced analytics solution must be able to receive it from the payment gateway and attach it to the sender and recipient.
Second, the solution must run the multiple “hops,” or connections, query on the connected data (graph). This real-time deep link traversal can include as many as six or more hops, starting with a user who initiates a payment and ending when a fraudulent payment transaction associated with a particular device is detected. And this process takes place in milliseconds.
Third, the advanced analytics should offer real-time computation and updates on the connected data. In other words, the solution should examine the trust score for a payment to see if it’s fraudulent. At the same time, the system should perform real-time updates; these include marking a new user as linked to potentially fraudulent accounts (“zero trust” score) or flagging an account that needs to be investigated before a payment can go through.
The “next best action” can be authorizing a payment or stopping a fraudulent payment depending on the outcome of deep link analysis and trust score computation.
Let’s take a look at advanced graph analytics in action across a number of industries.
Healthcare
When it comes to patient care, advanced graph analytics reveal the entire “patient journey to wellness,” examining multiple levels of relationships among interconnected data. This includes complex relationships among members, providers, facilities, and claims – in real-time.
“Multi-dimensional entity and pattern matching” is key; this refers to understanding the significance of a pattern of connections among different data types and searching for similar patterns across datasets. Healthcare providers can then answer questions, such as: “Are there members that have a similar journey to a specific member with a known medical condition?” We can look at age, gender, known medical conditions, and procedures to find matching patterns. Then, a healthcare provider or insurer can proactively call (the “next best action”) to offer support to a patient.
eCommerce
In today’s eCommerce realm, transaction speed is often the difference between a sale or no sale. Consumers also expect faster and more flexible payment methods.
Advanced graph analytics has an integral role in eCommerce – especially in the case of Pagantis, a point of sale consumer finance platform for eCommerce in Europe. Pagantis allows consumers to pay for goods and services in monthly installments with a fully automated, paperless process – with the help of advanced graph analytics. A consumer’s credit rating can be calculated in real-time, and a graph database is the only data model in which a customer’s data (and all the complex relationships among disparate pieces of data) can be analyzed for credit scoring, fraud detection, and risk analysis. Pagantis offers instant online approval, carried out in real-time through a scoring algorithm. A shopper is then given the choice to finance his purchase and presented with multiple offers.
Email Marketing for eCommerce/Retail
Speaking of consumers and eCommerce, let’s take a look at advanced graph analytics when it comes to real-time email marketing offers. A personalized shopping experience matters to consumers. A Gartner survey showed that 38 percent of customers will stop doing business with a company because of poor marketing personalization efforts.
Kickdynamic, an automated email marketing platform, uses advanced graph analytics to help its more than 200 global customers in fashion, retail, travel, and other sectors generate personalized and relevant email offers for customers. Kickdynamic taps into the power of advanced graph analytics to produce personalized customer emails based on demographics, user preferences, product features, location, browsing patterns, and purchase history. Regular analytics may only delve one or two hops into customer data, but more personalization is needed to foster brand loyalty. Advanced graph analytics enables Kickdynamic to go ten or more hops deeper and provide real-time deep link analytics that open up more information about a customer’s likes, intent, and needs. These live, automated, and personalized emails to customers are more likely to culminate in a purchase.
Understanding Impact, Taking Action
Advanced graph analytics allows us to take real-time information (orders, claims, payments), understand the impact of these particular transactions, and then figure out the next best action for a customer or organization – all in real-time. These next best actions can prevent fraud, generate additional product sales, or even offer critical support to a healthcare patient. These additional hops, connections, and deep link analyses are necessary to meet the demands of virtually everyone – from consumers to brands and everyone in between.