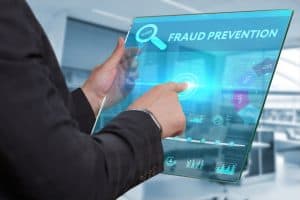
Advanced graph analytics provides deeper insights, complementing BI, and helps organizations preempt and prevent potential fraud while protecting customers.
Financial services organizations are both powerful and vulnerable. As a result, they are often a target for fraudsters and cybercriminals. Merchants and financial services organizations will spend $9.3 billion annually on fraud detection and prevention by 2022. Global online payment fraud (also called CNP or “Card Not Present” fraud) alone will cost merchants $130 billion in just five years (from 2018 to 2023). The latest report from LexisNexis also indicates that fraud attempts have increased significantly among financial services firms during the past year, with more than twice the number of attempts and an 85 percent increase in fraud success rates.
See also: AI Brings Real-Time to Fraud Detection and Prevention
As the scale and scope of fraud has increased to encompass digital and mobile customer platforms and devices, financial services organizations are placing “fraud prevention and detection” at the top of their priority lists. In fact, five of the ten largest global banks and two of the world’s largest payment card companies have turned to advanced analytics in graph for their anti-fraud initiatives. Gartner analysts have highlighted Graph Database & Analytics as a “top 10 trend for data and analytics,” with an estimated annual growth of 100 percent annually through 2022. This technology helps analyze, detect and visualize complex data patterns – patterns that indicate the potential for fraud.
Basically, graph analytics is a set of analytic techniques that allow you to “drill down” into complex interrelationships among organizations, people and transactions. For example, a leading U.S. multinational investment bank is using advanced graph analytics to improve its fraud avoidance initiatives, specifically fraud detection for debit and credit cards. The organization is adding graph analytics to its machine learning system to find data connections between “known fraud” credit card applications and new applications. As a result, the bank can identify more questionable patterns, expose fraud rings and shut down fraudulent cards faster. The bank will then save millions of dollars annually. Also, the world’s largest payment card provider chose advanced graph analytics for payment fraud detection and merchant credit management. The company, which had been using RDBMS (relational database management system), needed technology that offered deep pattern analytics and real-time update capabilities for its anti-fraud work.
Fraud detection requires understanding connections and identifying anomalies in links among people, transactions, payment methods, locations, devices, times and more — and working with huge and ever-changing datasets to do this in real time. The world’s most innovative financial services organizations are opting for advanced analytics in graph, or advanced analytics in connected datasets (“graph” refers to connected data). Advanced analytics in graph allows a system to process a payment while understanding how a transaction is connected to different datasets. Then, a “next best action” is offered up; in the case of fraud, this may be “reject payment” while marking a user with a “zero” trust score. But there is a significant difference between regular analytics and advanced analytics – especially when it comes to detecting fraud.
Fraud Detection for Financial Services With Regular Analytics
Let’s consider a typical payment via an online payment provider such as PayPal, Venmo, Apple or Samsung Pay to see an example of potential fraud and why it’s so difficult to detect with regular analytics.
A user, “User1,” has created a new account, Account 1, which is linked to their credit card from American Express. As a part of the setup and two-factor authentication, they have linked their phone number, Phone_number 1, and their email, Email 1, to their account. User1 is using an Apple iPhone 6 as their device with the registered phone number and initiates a payment, Payment 1, for $500 to Account 2. Up to this point, there aren’t any red flags or warnings in a traditional financial services fraud detection solution, because User 1 is a brand new user, with a new phone number and email (and none of these have been associated with any fraudulent transactions in the past with the payment service). Regular analytics does not find anything unusual or suspicious and the payment goes through without being flagged or rejected.
Financial Services Fraud Detection with Advanced Analytics Powered by a Native Parallel Graph Database
However, the deep link analysis with a native parallel graph database reveals a different picture — specifically, that — six layers in — there is fraudulent activity associated with a device, phone number and stolen credit card. Here’s how that unravels: The recipient account for the payment, Account 2, belongs to User 2, who has authenticated the account with Phone Number 2 as part of the account setup process. The phone number, Phone number 2, is used with Device 101, a Samsung Galaxy S3 device. As the deep link analysis digs through the history of prior fraudulent transactions and looks for devices associated with those transactions, it finds that the Device 101 was used last year with Phone Number 101 to set up Account 101. This account, Account 101, initiated a payment, Payment 101, which was later found to be fraudulent, as Account 101 was funded by a stolen credit card.
Advanced analytics with a graph database can go deep into the connected data, in this example, six hops, or connections, deep into the dataset to find the connection to prior fraud in real time and the payment transaction is rejected as a result.
As you can see, advanced analytics is needed for real-time fraud detection for payments – and advanced analytics detects fraud three levels “deeper” than what regular analytics is capable of. This gap between regular and advanced analytics can translate to hundreds of millions of dollars lost due to fraud. Advanced analytics with real-time can process the payment transaction in sub-second response time and then runs the multi-hop connections query on the connected dataset. In other words, the system must examine every link along the journey from User 1 initiating the payment (the first hop) to the final sixth hop, the fraudulent Payment 101. And this process is a fast one – up to 100,000 payments per second. The advanced analytics must also support “real-time computation,” such as looking at the trust score for a payment to see if it’s fraudulent. Only then can the next proper action be taken (“stop payment”).
Clearly, fraud detection is top-of-mind for every financial services organization – and this is unlikely to change. As fraudsters become more tech-savvy, it’s crucial that companies stay one step ahead of them. Advanced graph analytics enables these deeper insights, complementing existing BI technology and powering AI and machine learning. The result: organizations preempt and prevent potential fraud while protecting customers (and their hard-earned dollars).