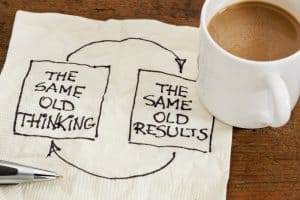
When we underestimate algorithms, entire industries crumble because they didn’t see change on the horizon. Case in point: Traditional taxi business vs. Uber.
As access to compute and data increase, algorithms will play a greater role in our lives, becoming a necessary component for business success. However, relying too heavily on algorithms, or using them in the wrong way, could be disastrous for businesses. For businesses to be successful in 2021 and beyond, they must develop an understanding of where algorithms can most effectively be used.
Understanding the limits
We’ve reached a point with AI where we simultaneously overestimate and underestimate the power of algorithms. When we overestimate them, we see human judgment relegated to an afterthought – a dangerous place to be. The use of a “mutant algorithm” in grading A-level results is the scandal du jour in the UK, despite the algorithm producing many results that simply violate common sense.
Sometimes when we overestimate algorithms, we anthropomorphize them. This is a mistake. Its axis of intelligence doesn’t map onto the same scale as that of humans. It doesn’t even have the same units. We can have algorithms that crush any human at chess but are unable to make a cup of tea and computer programs that can perform mathematics millions of times faster than humans but, if asked who might win the next World Cup, they wouldn’t even understand the question. Their capabilities are not universal.
See also: Researchers Surpass Speech Recognition Methods with Help From Honey Bees
When we underestimate algorithms, we see entire industries crumble because they didn’t see change on the horizon. How can the traditional taxi business compete when Uber’s algorithm can get you a ride in less than 3 minutes?
We know that for many enterprises, AI has taken over the first point of contact for customer service. They answer yes or no questions or can comprehend one or two-word answers to better understand the context of the request. But that’s where their involvement stops – once the complexity grows, consumers are directed to a real, human representative. That’s because companies have, over time, developed a good understanding of what AI can and can’t do.
Identifying the gaps
We live and conduct business in an increasingly globalized world. There are very few tech companies doing business only within the neighborhood or even the country they’re based in. If they’re going to adopt AI in the enterprise, they need to make sure that automated technologies – such as any-context speech recognition – can understand anyone regardless of their accent or dialect.
This is a tricky ask. Generally speaking, the accuracy of automatic speech recognition technology is favored toward American English. That means that when someone with a British or Australian accent interacts with a tool, it’s less likely to understand them than an American customer. This is also not easily visible; it’s a gap in the technology’s capability that relates to the back end but produces results that affect the user. As companies scale, this can be a real hindrance. So human understanding is needed here; understanding what AI is and isn’t capable of.
Finding the balance
The key to avoiding these blunders is to stop trying to map algorithms onto the human spectrum of competence. Algorithms simply don’t think like us. They’re not a machine that will eliminate the need for human employees. But they’re also a crucial part of the 21st-century tech stack and companies that don’t understand this risk being left behind.
It’s about finding the right balance between using AI when it’s needed and remembering that some jobs are better left to humans — or that sometimes, a job might be best accomplished with humans and AI working alongside each other. Looking to 2021, the ability to make this distinction will be crucial to business success.