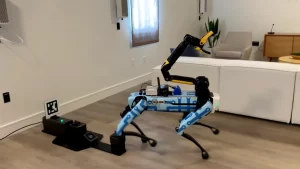
Facebook parent company Meta has published two advancements in the field of AI robot movement, based on copying human interactions.
Meta Platforms, the parent company of Facebook, announced two advancements in the field of robotic artificial intelligence, aimed at improving sensorimotor skills in a robot.
The first advancement is an artificial visual cortex; a single perception model which can understand and replicate a range of sensorimotor skills. The model has been trained on the Ego4D dataset, developed by Meta AI in partnership with several academic partners, which has thousands of videos of humans performing everyday tasks. Meta has announced it will be open sourcing the dataset, so other robotics teams can utilize it.
SEE ALSO: AI and Robotics Research Continues to Accelerate
The visual cortex tries to replicate the region of the human brain which converts vision into movement. In this instance, the AI converts camera inputs from humans into robotic actions. This is can do even though the robot is not built the same as a human – during testing Meta used Boston Dynamics Spot robot.
“Although prior work has focused on a small set of robotic tasks, a visual cortex for embodied AI should work well for a diverse set of sensorimotor tasks in diverse environments across diverse embodiments,” said Akshara Rai, research scientist at Meta. “Our results show VC-1 representations match or outperform learning from scratch on all 17 tasks. We also find that adapting VC-1 on task-relevant data results in it becoming competitive with or outperforming best-known results on all tasks in CortexBench.”
The second advancement is a new approach to robotic mobile manipulation, called adaptive sensorimotor skill coordination. Meta claims this new approach is far better than previous methods, with 98 percent success on tasks such as navigation, picking up an object, placing the object, and repeating processes.
Meta trained the robot entirely through simulation, which removes the rigidity that real-world testing can sometimes cause. Instead of faltering at the first change in the real-world, a robot trained with sensor-to-action neural networks is more capable of adapting to changes.
“When we put our work to the test, we used two significantly different real-world environments where Spot was asked to rearrange a variety of objects,” said Rai. “Overall, ASC achieved near-perfect performance, succeeding on 59 of 60 episodes, overcoming hardware instabilities, picking failures, and adversarial disturbances like moving obstacles or blocked paths. In comparison, traditional baselines like task and motion planning succeed in only 73 percent of cases, because of an inability to recover from real-world disturbances.”
Part of the issue with simulation to reality transfers in the past has been that developers attempt to replicate all of the physical attributes of the real-world inside the simulation. Meta, in its latest test, used a counterintuitive approach in simulation by focusing on the high-level policy ideas such as where to go rather than low-level physics, such as how to move the legs.
While science fiction writers and technologists of the 20th century expected the physical attributes of robots would be solved way before the mental, the opposite looks to be true. With ChatGPT and other generative systems able to trick humans into believing they are real, we seem to be on the cusp of AI which is creative, artistic, and fun, but we still appear to be a few generations away from robots that can do even the most basic tasks as well as humans.