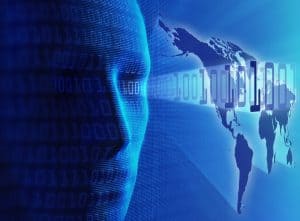
NIST makes a strong argument for looking beyond data and even the ML processes to discover and destroy AI bias
By now, no one should dispute that most AIs are built upon—and currently wield—a certain degree of problematic bias. It’s a challenge that’s been observed and confirmed hundreds of times over. The challenge is for organizations to root out AI bias and not be satisfied with simply pushing for better, unbiased data.
In a major revision to their publication, Towards a Standard for Identifying and Managing Bias in Artificial Intelligence (NIST Special Publication 1270), after a public comment period last year, the National Institute of Standards and Technology (NIST) makes a strong argument for looking beyond data and even the ML processes to discover and destroy AI bias.
Instead of blaming poorly collected or labeled data, the authors say the next frontier in AI bias is the “human and systemic institutional and societal factors,” and push for a social-technical perspective toward finding better answers.
“Context is everything,” said Reva Schwartz, principal investigator for AI bias at NIST and one of the report’s authors. “AI systems do not operate in isolation. They help people make decisions that directly affect other people’s lives. If we are to develop trustworthy AI systems, we need to consider all the factors that can chip away at the public’s trust in AI. Many of these factors go beyond the technology itself to the impacts of the technology, and the comments we received from a wide range of people and organizations emphasized this point.”
What are these human and systemic biases that contributed to AI bias?
According to the NIST report, human bases come in two broad categories: individual and group, with numerous specific biases beneath each.
Individual human biases include automation complacency, where people over-rely on automated skills; implicit bias, an unconscious belief, attitude, associate, or stereotype that affects the way someone makes decisions; and confirmation bias, which is when people prefer information that aligns with or confirms their existing beliefs.
Group human bases include groupthink, the phenomenon of people making less-than-optimal decisions based on the desire to conform to a group or avoid dissension; and funding bias, when biased results are reported to satisfy a funding agency or financial supporter, which could in turn be influenced by additional individual/group biases.
And for systemic biases, the NIST report defines them as historical, societal, and institutional. Essentially, the long-standing biases that have been encoded into society and institutions over time and are largely accepted as “fact” or “just the way things are.”
These biases matter because of how influential AI deployments are in the way organizations work today. People are denied a mortgage loan, robbing them the opportunity to become a first-time homeowner, because of racially-biased data. Job-seekers are denied job interviews because AIs are trained on historical hiring decisions, which favored men over women. Promising young students are denied interviews or acceptances at universities based on their last names, which don’t match up with the names of those who have been successful in the past.
In other words: biased AI creates as many locked doors as it does efficiency openings. If organizations don’t actively work to cut bias from their deployments, they’ll soon find a disastrous lack of trust in how they think and operate.
What is the socio-technical perspective that NIST recommends?
At its core, it’s the recognition that any AI application results from more than mathematical and computation inputs. They’re made by developers or data scientists, in many different positions and institutions, all of which carry a degree of baggage.
“A socio-technical approach to AI takes into account the values and behavior modeled from the datasets, the humans who interact with them, and the complex organizational factors that go into their commission, design, development, and ultimate deployment,” reads the NIST report.
By taking a socio-technical perspective, argues NIST, organizations can do a whole lot more to cultivate trust through “accuracy, explainability and interpretability, privacy, reliability, robustness, safety, and security resilience.”
One of their recommendations is for organizations to implement or improve their test, evaluation, validation, and verification (TEVV) processes. There should be ways to mathematically verify the bias in a given dataset or trained model. They also recommend creating more participation, from a wide variety of fields and positions, into AI development work, and having multiple stakeholders from different parts—or outside—the organization. “Human in the loop” models, where a person or collective constantly corrects the foundational ML outputs, are also an effective tool against bias.
Beyond those and the revised report, there is NIST’s Artificial Intelligence Risk Management Framework (AI RMF), which is a consensus-driven set of recommendations to manage the risks involved in AI systems. Once completed, it will cover transparency, design and development, governance, and testing of AI technologies and products. The initial comment period for the AI RMF has passed, but there are still plenty of opportunities to learn about AI risk and the mitigations that work today.