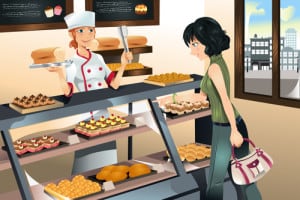
How artificial intelligence and analytics can be used to better manage perishable inventory.
Name of Organization: Bakkersland
Industry: Food
Location: Hedel, The Netherlands
Opportunity or Challenge:
Bakkersland, the largest baking company in the Netherlands, needed to get a better idea of what demand for its products would be on a day-to-day basis, so it could produce accordingly. The company, which has 300 trucks delivering 2 million fresh baked breads to 1,200 supermarkets every day, needed to reduce the amount of leftover products left on the shelves.
As the leftover products can’t be sold the day after, retailers have the tendency to order less than they expect to sell. Product unavailability is a large cause of dissatisfaction among customers, which often goes unnoticed by the retailer. As documented in a recent case study, Bakkersland wanted to use information technology and artificial intelligence to optimize turnover and service levels.
“Retailers tend to order their bread products just in time, and during the production process we still regularly receive changes,” according to Hein Boersma, ICT manager at Bakkersland. “In the remaining production time, we need to produce the exact numbers up to our quality standards. Not only do we have to deal with changes in demand, fluctuations in the production process, caused by numerous factors, occur regularly as well.”
Meeting the Challenge:
To predict the consumer demand per-day and per-retailer, Bakkersland asked GoDataDriven, a division of Xebia Group, to develop a predictive sales planning model. This machine learning model optimizes the availability of fresh bread products, while at the same time minimizes left-over.
The team developed an artificial intelligence model that determines the ideal amount of fresh bread products per supermarket per day over a longer period by analyzing transaction details combined with external data. The model provides Bakkersland with the right data to automatically produce the ideal amount of bread for every supermarket, based on demand and the specific assortment preferences of the retailer. The new system provides insight in predicted demand over longer periods of time. Once the production need is known upfront, the bakery can suggest promotion slots to retailers, and thus stimulate demand in times of overcapacity.
To improve data quality, the team also implemented a data cleaning process intended to filter mistakes made at the cash register, such as transactions of large amounts of bread that are flushed immediately after they have been sold. Also, the process matches retailers’ own codes with Bakkersland’s product codes.
As the model recognizes patterns, feedback from the retailers is crucial in training the model. By combining input from retailers and the increasing amount of data, the model’s predictions become more accurate, which in turn leads to retailers building confidence and trust in the model.
Benefits From This Initiative:
After going live at one supermarket, the new system and machine learning model lead to a decrease of sold-out products and the minimization of leftover products, the case study reports.
Ability to address individual customer requests also has been heightened. For instance, on one given Wednesday, a customer was unable to find her type of bread. After carefully studying the data, it turned out that this customer was the only person who bought this type of bread, and she always came in on Thursdays. Now, the supermarket manager and the customer discussed that when she plans to come to the store on a different day, she contacts the supermarket beforehand, so they can order her preferred type of bread.
Results from the model’s first full implementation period are positive, the case study reports, with visibly improved sales figures and product availability. The company plans to connect all its supermarkets into this intelligent, real-time demand planning system.
The company is also working to use sales analysis to include profit margins in the model as well. By using this data as a feature in the model, it will become possible to optimize the profit per retailer by steering on the availability of specific bread types.