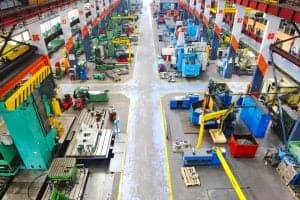
Manufacturing has a very broad set of use cases for artificial intelligence including predictive maintenance, quality control, price forecasting, and more.
The potential of artificial intelligence in manufacturing is so limitless that it’s difficult to know where to start. AI is used to perform manufacturing and quality control, and can shorten design time, reduce materials waste, improve production and perform predictive maintenance.

The power of artificial intelligence is explored in a recent eBook, published by Sinequa, which explores the obstacles to fully leveraging AI in manufacturing, and steps to overcome them. “It’s like having a Lego set. Rather than having to build a house from scratch, you’re now building a house out of a Lego set which is a lot easier.” However, obstacles preventing AI deployments in manufacturing is “the same story as with other verticals – a lack of knowledge and qualified personnel.” the book’s authors state. “This problem is compounded by a lack of budget to fix the problem – investments in any new technology will inevitably lead to questions about ROI. And this is something that’s hard to estimate without proofs of concept already in place.”
The book outlines five proven applications for artificial intelligence in manufacturing:
Automated quality checks: “Computer vision systems have a superhuman ability to identify rapid defects that even experienced employees would miss. The machines will either ensure they’re rejected, or alert an engineer for a second opinion. The result? More reliable products, and less time and money spent on costly repairs.”
Predictive maintenance: “While engineering and materials have come a long way, the problem of machines breaking down and needing repair is ever-present. This is not something that can be done by just looking at machinery. It typically involves a whole host of sensors analyzing everything from acoustics to thermal performance. Machine learning models can, for example, detect possible misalignment, wear, and tear, or looseness in rotating machinery, by monitoring vibrations the equipment emits.”
Data collection and intelligent search: “Companies are sitting on mountains of data that are essential for everything from fruitful R&D to the smooth running of actual production lines. The trouble is that this data can go back decades, is often stored across multiple locations, and in hundreds of different versions and formats. More importantly, such data is largely unstructured, making it incredibly difficult to collect, process, and analyze. Intelligent search can process massive amounts of raw unstructured data – everything from emails and notes to CAD designs and issue logs – with greater accuracy than ever before. Intelligent Search technologies use AI to access, enrich, and unify content and data wherever it lives.”
Generative design: “A designer, no matter how creative, can only come up with so many ideas every day. With AI and generative design, the sky’s the limit. Designers can input the type and quantity of materials needed, the manufacturing process required, and their budgetary constraints into generative design software. Machine learning models can then produce several options.”
Price forecasting: “If your products are made from materials with fluctuating prices, AI-based systems can be of enormous help. They can advise when to buy, and estimate the exact cost of each item rolling off the production line.
Think of it as the dynamic pricing algorithms e-commerce giants like Amazon use, but in reverse. For dynamic pricing, companies analyze the prices charged by competitors, as well as historical data, to ensure their prices are always appropriate..”
The book also takes a deep dive into AI-driven work at BAE Systems, a British aerospace manufacturer. The company “created a testbed for innovative new systems called Factory of the Future, a blend of ‘proof of concept’ ideas and emerging technologies that are being proposed around the world. ‘It’s intended to be something that drives innovation forward, drives partnership and collaboration, and allows us to explore very low maturity, novel ideas,” said Austin Cook, principal technologist for emerging technology and systems at BAE. “But it’s also supposed to be a vehicle through which we bring new capabilities, technology, and skills through at a pace and where we can exploit them now for business benefit and value.’”
