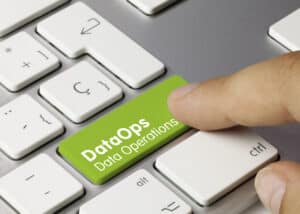
Organizations can leverage the inherent agility of DataOps to drive innovation, enhance operations, and make confident data-driven decisions.
DataOps, which aims to streamline and automate end-to-end data pipeline operations, has gained prominence in recent years because of its ability to create an agile strategy. Combining best practices from DevOps and data governance, DataOps delivers high-quality data with improved security and compliance and fosters a culture of collaboration and continuous improvement among data citizens, IT, and business teams. But many organizations still struggle with the concept and how to get started.
Fundamentals
DataOps involves automating data collection and analysis in an effort to increase reusability, democratize data access, and continuously monitor the data pipeline. DataOps ensures valuable metrics are identified, relevant data sets are utilized, and technologies are applied to extract, transform, and analyze data.
Key principles include:
- Collaboration: Brings together data engineers, data scientists, and DevOps engineers to ensure that everyone is working towards the same goal and that data is shared and used effectively. Allows for the leveraging of diverse perspectives and skills and leads to more robust data solutions and informed decision-making.
- Automation: Automates as much of the data lifecycle as possible to improve the speed and accuracy of data processing and reduce the risk of human error. Automated data processes accelerate time-to-market by enabling faster data delivery, empowering individuals to generate insights and make data-driven decisions more quickly. It also facilitates scalability by allowing organizations to handle large volumes of data without compromising performance, ensuring flexible scalability as data needs continue to grow.
- Continuous improvement: Constantly monitors and improves processes to ensure that DataOps is optimized and adaptable to evolving business requirements.
See also: DataOps: How to Turn Data into Actionable Insights
Contrasting DataOps with Traditional Data Management Approaches
Conventional data management approaches tend to be siloed and fragmented, which leads to data quality issues, slow time to market for data-driven insights, and increased operational costs. As a result, these data management practices tend to struggle with manual efforts, and their lack of automation leads to slow and inefficient data delivery. Further, the lack of data quality and governance issues slows down the process of onboarding new data users. DataOps, on the other hand, is a more comprehensive style of data management. It brings together business stakeholders, allowing them to work together and automate the data lifecycle and ensures that data is always available, up-to-date, and of high quality and used by technical and business stakeholders across the enterprise.
The key components of a DataOps lifecycle include:
- Data ingestion: Involves loading data into a data warehouse or data lake. This can be a complex process, as data may come from a variety of sources, such as operational systems, transactional databases, and external sources.
- Data curation: This is the process of cleaning, transforming, and enriching data. This helps to ensure that data is of high quality and ready for analysis.
- Data observation: Monitors data quality and performance. This helps to identify any potential issues with the data, such as data drift or data corruption.
The Benefits of DataOps
Achieving faster time-to-market for data-driven solutions enables organizations to accelerate the time-to-market for data-driven solutions. Because DataOps helps to automate the data lifecycle – from data collection to analysis to deployment – it frees up data scientists and other business users to focus on more strategic tasks, such as developing new insights and generating new business opportunities. It also improves data quality and governance by facilitating effective management of the data lifecycle, which ensures that data is consistently available, up-to-date, and of high quality.
Finally, operational costs are reduced by automating data processes and eliminating manual tasks. This improves operational efficiency, minimizes human errors, and optimizes resources for higher-value activities. Automation enables scalable data operations, accommodating growing data volumes without significant cost increases, and allows organizations to manage larger datasets and deliver timely insights without incurring substantial expenses.
See also: A Good Data Analytics Program Relies on Good DataOps
How to Begin: Best Practices for a Successful Implementation
Implementing DataOps requires a thoughtful and systematic approach. To get started, enterprises need to assess the current data landscape to understand the existing data management processes, systems, and pain points within their organization and identify areas where DataOps can bring the most value. Companies must also set achievable goals and priorities by defining clear objectives for why they are implementing a DataOps strategy. Start with specific use cases or projects that can demonstrate the benefits to stakeholders and then gradually expand the scope to cover broader data operations.
To ensure a successful DataOps implementation, consider the following best practices:
- Build a skilled and collaborative DataOps team: Assemble a cross-functional team with expertise in data engineering, data science, and DevOps. Encourage collaboration and provide opportunities for continuous learning and skill development.
- Establish a robust data governance framework: Define data governance policies, standards, and guidelines to ensure data quality, security, and compliance. Implement data cataloging and metadata management practices for improved data discoverability.
- Leverage cloud-based technologies for scalability and flexibility: Cloud platforms offer scalability, flexibility, and cost-effectiveness for DataOps implementations. Utilize cloud-native data services, infrastructure, and analytics tools to leverage their capabilities.
- Continuously monitor and optimize DataOps processes: Regularly evaluate and refine DataOps processes to identify bottlenecks, improve efficiency, and enhance data quality. Implement performance monitoring and analytics to gain insights into data operations.
DataOps enable organizations to overcome data management challenges and make rapid business decisions. It also unlocks the full potential of data, providing a competitive advantage in the data-driven era, allowing organizations to experience faster time-to-market, improved data quality and governance, increased collaboration, and reduced operational costs. However, implementing DataOps requires a combination of effective processes, automation, and a collaborative culture that aligns both IT and business teams as well as data citizens. With continuous improvement and the right strategies in place, organizations can leverage the inherent agility of DataOps and apply it to drive innovation, enhance operations, and make confident data-driven decisions.