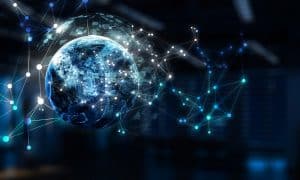
AI can unlocked the hidden knowledge in the vast amount of data in backhaul networks, making it available for troubleshooting and to improve network performance.
Behind the mobile access radio network lies an intricate transport network that, like a vascular system, reaches all the access nodes with a capillary spread out across the country, ensuring population and terrain coverage. With every new generation of mobile access technology, the number of access nodes kept growing, and with 5G is foreseen a new densification coverage. Every new wave brought additional services as well as challenges. Backhaul networks evolved accordingly, encompassing advanced functionalities to satisfy and, in most cases, outperform the new needs.
According to the GSMA and ABI Research report 2021[Ref1], mobile backhaul networks globally are mainly served by microwave radio links connecting over 60% of the global base stations and by fiber optics for the remaining part. Furthermore, the use of microwave and millimeter-wave radio is forecasted to remain the dominant technology for the next six years at least, representing the time frame of the report’s study.
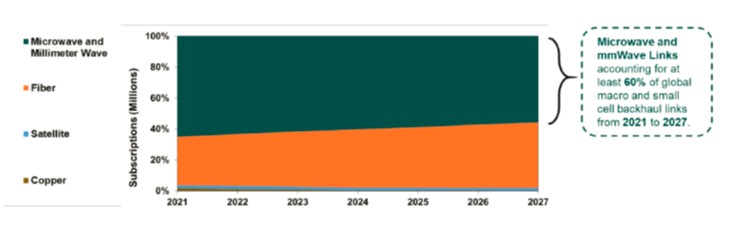
It is also known that excluding fiber-rich countries (like Japan, Korea, and China), the percentage of microwave adoption radio grows to surpass the 70% mark.
Parallel to the product evolution, the management and supervision of these technologies also become more and more articulated, including more monitoring points and alarms as the product configurations became elaborated, with the result of weighting on the operations activities of service set up but more importantly on maintenance and troubleshooting.
Generating and managing big data
A single microwave radio link, in the course of a year generates a multitude of data related to alarms, traffic, and propagation monitoring points. Initially, when microwave radios were simple capacity pipes, most of the data was related to the propagation layer. Nowadays, a microwave radio includes Carrier Ethernet fabric, ip/mpls service engine, packet synch, and nodal configurations leading to multiple radio channels all associated with a single network element. This has vastly augmented the quantity of generated data. An average European mobile backhaul network comprises 20,000 to 30,000 in service links, generating over 1 million alarm events daily, plus performance indicator monitoring points that lead to a staggering estimated 1 to 2 billion records yearly.
To reduce risks of vendor lock-in and monopoly, mobile operators generally adopt a multi-vendor sourcing strategy. This introduces a further level of complexity as today each vendor uses proprietary protocols to read, classify and store monitoring equipment data.
The generated big data is a collection of records that shows the health status and connectivity behavior of the entire network. On the one hand, this offers great knowledge to the operation team that can tap into this data to retrace the events from the “standard” operational behavior to the moment an impairment took place. On the other hand, the wide amount of data makes it daunting to address and time-consuming to carry out, two aspects that during the pressure of a traffic effecting event do not help accomplish a swift and cost-effective resolution of the crisis.
These aspects are driving many operators to run in a reactive mode. As an event occurs on a specific node, link, or portion of a network, the relative historical data is retrieved and analyzed to resolve the issue at hand as fast as possible. This poses several behavior considerations; once an event occurs, the operation team needs to run in “critical mode” as the longer the traffic remains interrupted, the higher the generated losses. The time pressure pushes to act quickly and may lead to multiple parallel activities resulting in higher expenditure costs, like unnecessary site visits.
Analyzing backhaul networks data with AI
Considering the vast amount of data produced by the wireless backhaul network, using an artificial intelligence application to analyze it represents the most effective way to make sense of such a large data quantity. Using deep learning and neural network training, the AI-driven app can learn to identify:
- data correlation between the propagation channel and the generated alarms,
- network behavioral trends that may lead to congestion or traffic loss
- learn how and when data peaks are expected due to network busy hours and how to remove the bottlenecks
These activities can be performed in seconds, resulting in clear pointers to where the network needs to be addressed and how. Saving time, acting on specifics and localized items, and reducing to the minimum expenditures.
A technical article about how a neural network has been set up and trained to achieve these results can be found in this IEEE article titled “supervised and semi-supervised learning for failure identification in microwave networks.” paper.[ref2]
With this holistic approach, the AI application can carry out data gathering and analysis, offering continuous feedback on the network health status. Furthermore, with the implementation of the prediction algorithm, the app can point to where a bottleneck or a possible impairment may take place in the future. This allows for the operational team to timely plan in-field operations maximizing the network up-time and reducing chances of unexpected traffic interrupting events, striving for an “always-on” infrastructure.
A new operational model
Thanks to AI, the tremendous knowledge hidden in the big data is unlocked and at anyone’s disposal in a click. This can now be accessed not only as a troubleshooting tool but to open up new activities continuously improving the network performances, allowing the engineers to focus on the solution and not on tedious data analysis. The AI-based application offers great and unparalleled insight to the maintenance and operation team, who can now shift their operational mode to a preventing one:
- Immediately pinpoint the fault root cause of an event and its relationship with the network: is it a propagation issue (e.g., interferences or adverse propagation conditions), is it a data layer issue (e.g., switching or routing), is it due to external factor not related to the backhaul (e.g., missing synch source or loss of power)
- Drastically reducing go-to-field costs, as the AI would offer a higher accuracy in determining the fault root cause and if this needs field intervention.
- It can put in place automated responses or immediately offer the operator how to fix the issue at hand, cutting down the response time, resulting in a much higher network uptime.
- It can predict the “traffic growth trend” to test the network resilience, identifying where and how to upgrade the infrastructure for higher robustness, preventing possible future outages. This offers the opportunity to plan any equipment upgrade that may be needed during optimal network operation and not during a crisis situation due to the weakness of a specific link.
AI/ML applications have proven to offer great flexibility and deep network knowledge. Thanks to an SDN workframe, an operator is also relieved from the multi-vendor data collection differences based on proprietary OSS solutions that are now equalized by the adopted standard models. This ensures that the data type and quality remain consistent throughout the network, regardless of geographical dislocation or technology provider.
This technology offers great advantages addressing the whole network seamlessly cross-vendor. In perspective, it minimizes the times impairments hit the network, maximizing its monetization due to higher service up-time. The result is a higher connection reliability, fitting for 5G critical services, resulting in a more accost effective running of the network.
[Ref 1] Wireless Backhaul Evolution – February 2021 – GSMA & ABI research
[Ref2] IEEE-supervised and semi-supervised learning for failure identification in microwave networks