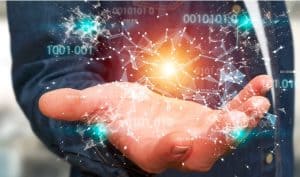
Combining the digital twin concept and the power of in-memory computing promises to offer compelling real-time solutions.
We are increasingly surrounded by intelligent and chatty devices. With the rapid adoption of IoT, now numbering more than 21 billion devices, telemetry streams are inundating cloud-based servers with incoming messages. These messages must be analyzed quickly to identify potential problems or uncover elusive opportunities. Software that implements intelligent real-time monitoring and analytics must evolve beyond current techniques and provide better answers faster to tackle this problem and help managers maximize their situational awareness.
Examples of software for real-time analytics abound, including telematics software that tracks vehicles in a fleet, security software monitoring physical points of entry or network endpoints in a cyber infrastructure, health-tracking systems that analyze telemetry from wearable devices, fraud-detection software for credit card transactions, and many others. These applications are tasked with the need to simultaneously digest messages from numerous data sources, find patterns of interest, and act quickly when necessary.

Digital Twins: A Key Enabler for Real-Time Analytics
The digital twin model can help address this challenge. This model has evolved over the last twenty years for describing the state and behavior of devices, and it has been widely adopted in the field of product lifecycle management (PLM). Its object-oriented design provides a convenient means for encapsulating the properties of a device and a model of its behavior. Instead of just using these capabilities to assist in the design process, digital twins can be employed to process telemetry for real-time analytics. Using digital twins in this way offers a powerful means to track the dynamic state of a physical device and identify unusual changes that require attention. It also has the potential to simplify application design for streaming analytics algorithms.
Consider the leverage that digital twins can create for monitoring a complex, live system with numerous data sources, such as a fleet with thousands of trucks. Conventional real-time monitoring systems ingest and store telemetry in a log file or database and periodically query the data store to look for anomalies and trends in the telemetry. Message data often may be migrated to a data lake for offline analysis using big data techniques. This architecture introduces delays ranging from minutes to hours and can miss real-time issues that are hidden in the torrents of incoming telemetry. In contrast, digital twins can immediately process telemetry from each data source using sophisticated techniques, such as predictive analytics and machine learning (ML), and they can alert personnel to emerging issues within a few milliseconds.
Implementing Real-Time Analytics at Scale with In-Memory Computing
In-memory computing platforms have evolved over the last two decades to provide highly scalable, memory-based storage with integrated computing that spans a cluster of physical or virtual servers. Designed to store and process object-oriented data, they offer an ideal platform for hosting thousands (or even millions) of digital twins and running their analytics algorithms. The ScaleOut Digital Twin Streaming Service is an example of an in-memory computing platform designed for this purpose.
To harness the power of in-memory computing for streaming analytics, the platform stores each digital twin as an in-memory object, one digital twin for each physical data source. It directs incoming messages from data sources to their respective digital twins and processes each message immediately after it arrives. Message processing uses analytics code and ML as needed to update the digital twin’s properties and determine whether to send an alert.
Because of its inherent speed and scalability, in-memory computing can ensure consistently fast analysis measured in milliseconds, even for large workloads, and it can simultaneously process telemetry for thousands of data sources. In addition, it can incorporate data-parallel computing techniques that continuously aggregate properties of all digital twins so that personnel can track and visualize strategic changes within seconds. For example, telematics software for a trucking fleet can identify a regional delay affecting a group of trucks and visualize how the delay is spreading over time.
The following diagram illustrates how in-memory computing with digital twins can accelerate message processing compared to conventional techniques and provide real-time results in milliseconds:
Integrating Streaming Analytics with Traditional Digital Twin Models
Popular cloud-based digital twin platforms, such as Microsoft’s Azure Digital Twins, offer comprehensive features for asset tracking, including specialized modeling techniques and visual display tools. Integrating Azure Digital Twins or similar platforms with scalable, in-memory computing can expand their range of applications from PLM to real-time analytics for live systems with many data sources.
This integration can be accomplished by selecting a subset of digital twin properties to be used by in-memory computing in message processing. For each Azure digital twin, the in-memory platform retrieves these digital twin properties on startup, hosts them in an in-memory object for fast access, and analyzes incoming messages. When it updates properties, it persists these changes back to the corresponding Azure digital twin. This integration provides a powerful execution platform for real-time analytics with integrated ML and offloads latency and scaling requirements, as illustrated below for the Azure Digital Twins service:
Summing Up: Digital Twins Enable Real-Time Analytics at Scale
With our ever-growing dependence on complex, live systems that provide essential services, real-time analytics has taken on an increasingly important role in ensuring smooth, uninterrupted operations. It has become infeasible for personnel to monitor telemetry from the huge number of data sources they must track, and existing analytics techniques typically do not provide the fine-grained introspection required for real-time responsiveness.
Combining the digital twin concept and the power of in-memory computing promises to offer compelling real-time solutions to this challenge. These technologies enable real-time systems to deliver the detailed analysis of incoming telemetry required to immediately identify emerging issues or capture elusive opportunities. In-memory computing can easily be integrated into existing digital twin platforms, such as Azure Digital Twins, to unlock new use cases that take them beyond PLM to tackling the daunting task of effectively managing today’s mission-critical, live systems.