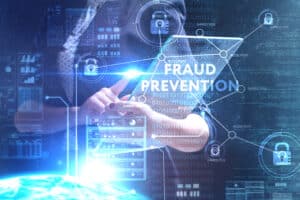
To stay a step ahead of the bad guys, businesses are leveraging artificial intelligence (AI)-powered automation to identify patterns of suspicious activity and thwart issues that can cause organizational, financial, and reputational harm.
It’s an unfortunate reality that economic uncertainty ignites fraudulent activity. According to the Nations on Occupational Fraud and Abuse, organizations lose 5% of revenue to fraud each year, totaling more than $4.7 trillion lost to fraud globally. That number is expected to continue climbing as desperation fuels criminal activity in the face of a vulnerable economy challenged by a looming recession, interest rate hikes, and bank volatility.
To stay a step ahead of the bad guys, businesses are leveraging artificial intelligence (AI)-powered automation, including machine learning (ML) and automated decisioning, to identify patterns of suspicious activity and thwart issues that can cause organizational, financial, and reputational harm.
Why AI is Key in Helping Fight Fraud
As economic volatility persists, it is crucial for any organization to bolster its fraud management and mitigation approach. AI can provide fast, accurate, and cost-effective defense that not only helps detect fraud in real time but can also prevent it from happening in the first place —all without compromising the customer experience.
By leveraging ML, businesses can continuously monitor systems and compare hundreds of thousands of data points to spot abnormalities and detect “red flag” patterns within a matter of seconds. They can also adapt and learn from new fraud patterns and trends, improving detection over time.
In addition to detecting fraud, AI optimizes the detection of fraud by automating repetitive tasks, such as reviewing transactions or verifying identities and triggering steps to stop or prevent fraud—reducing the time and resources needed for tedious manual intervention or mitigation.
For example, if a bank’s ML algorithm detects fraud in a credit card transaction, AI can prompt a series of actions, such as notifying the card owner that it’s being handled, prompting the card owner to confirm the validity of the transaction, canceling and reissuing credit cards, or alerting credit reporting agencies. By automating fraud detection and response with AI, this bank can mitigate the financial impact on the card issuer and the business. Additionally, it can minimize the inconvenience to the customer and minimize time spent by bank team members on manual intervention.
See also: Mortgage Fraud AI Improves Detection By 30%
Best Practices for Using AI to Stop or Prevent Fraud
Leverage a Comprehensive AI Approach
Businesses across industries, especially financial, insurance, or retail companies that process thousands of daily transactions, need to strike a balance between the intended goal of preventing fraud losses versus preventing false-positive alerts. In order to do that, businesses should pair the digital twins of supervised, explainable AI models with unsupervised AI models.
Explainable AI models can flag suspicious activity based on all previous transactions and give detailed, updated user and behavior breakdowns to continually hone fraud profiles and increase detection accuracy. Other types of AI models can flag transaction anomalies regardless of prior activity, enabling immediate detection of never-before-seen fraud attacks.
Using a comprehensive approach can take fraud detection to another level and allow for more accurate and real-time analysis that the business can leverage to both detect and prevent fraud.
The Power of Automated Decisioning
While prevention is best, if fraud does occur, it’s imperative to take immediate action to stop it in its tracks or, if it’s already taken place, mitigate any fallout and remedy the issue for the future. Using automated decisioning, logic-based decisions can trigger the appropriate next actions, such as declining the transaction, routing to a specialist, and more. Decision logic can provide valuable guardrails and human-driven policy (e.g., business rules) to overcome short-term inaccuracies in a decision model. Automated decisions can also be deployed on the front end of transactions to stop fraud, such as automatically declining known stolen credit card numbers.
Augment Human Intelligence, Don’t Replace It
Many view AI as a replacement for human intelligence instead of for the amplification of it. Keeping humans in the decision-making loop decreases the likelihood of unintended consequences or undesirable outcomes. By having trained employees oversee, manage, and train models, it becomes easier to mitigate ethical concerns regarding bias in AI deployments. Additionally, this approach enhances the performance and transparency of the models, ultimately boosting trust and confidence in the algorithms’ output while eliminating potentially inaccurate results that can disrupt operations or tarnish reputations.
See also: Next-Gen Online Fraud Detection: The Journey is the Destination
Staying A Step Ahead of Scammers
As economic uncertainty lingers, businesses must boost their efforts to detect and prevent fraud. AI systems, including machine learning and automated decisioning, provide businesses with an accurate, immediate, and cost-effective way to defend against fraud while minimizing the impact on the customer experience. While certain elements and risks of fraud cannot be eliminated, the combination of machine learning and rules give businesses the leverage they need to stay one step ahead of scam artists.