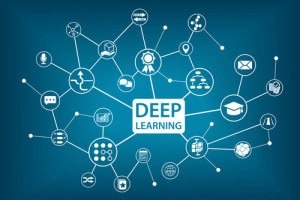
To solve real-world problems with AI, a deep learning system would need to be trained on a trillion parameters in 20 minutes. That may happen soon.
Even Intel is willing to admit that computers are great at crunching numbers, but not so great that they also make good decision-makers. Based on a recent webinar about the hardware advancements that have made better artificial intelligence (AI) possible, and what the future holds, that is about to change, and much faster than many would believe.
Pradeep Dubey, the director of the Parallel Computing Lab at Intel, explained the difference between traditional AI systems and newer implementations like deep learning—primarily, it’s about who is making the rules. In traditional AI, humans have to create rule-based systems for understanding which data should be processed, and how. This can take up an inordinate amount of time, and often, people don’t even know exactly what they’re looking for in the first place.
Deep learning, on the other hand, presents an algorithm with a set of data and allows it to find the rules itself. By training—in order words, cycling through sense, reason, action, and adaptation a countless number of times—deep learning algorithms can deliver better-than-human performance in many applications. Deep learning already being used in situations like automated driving, but it’s not the execution that’s being held back by hardware. It’s the training itself.
Deep learning challenges
Because deep learning efficacy scales with more data, there’s strong incentive to use millions of hours of video data, for example, to build out an automated vehicle system. This amount of data takes exaflops of computing power to be transformed into proper training, and according to Dubey, one can’t just throw more nodes at the problem.
Why not? In deep learning, scaling is “I/O bound”—in other words, the processors are fast enough to handle the data, but can’t pull data off the disks fast enough. By making training less communication-bound, he says, there’s potential for big jumps in throughput, but none of this is easy work.
To compound the issue, computing power is only a small portion of the actual trouble that prevents more deep learning advancements. Dubey says that 95 percent or more time is taken up by data management tasks. For most companies, between the hurdles of data management and the need for raw computing power, the challenges are simply too great.
The future is going to get deeper
For those who want to jump headfirst into deep learning, but can’t invest to heavily in infrastructure, the future is still bright. Dubey says, “I’ve never seen research move any faster—literally hitting the road—than with what’s happening in the world of deep learning.”
The innovations are happening on a number of levels, including algorithmic evolution, which will help deep learning to do more with less, and without as much supervision. More efficient deployments will help as well, as there are tradeoffs between throughput, accuracy, and model size, and not every problem will use the same parameters.
Intel itself is releasing a bevy of new processors that are not only faster than previous iterations, but also more optimized for deep learning. Dubey says the company is putting much of its effort behind new Xeon processors, but the Lake Crest architecture also offers true model parallelism, the capability to process tensors, and inter-chip links that are supposedly 20x faster than PCI Express.
Dubey is nothing but bullish on the future: “If you look at deep learning … the performance has improved almost 60-, 70-fold over the last three years only. Things that used to take 60 days are now happening in one day of training time. We want to scale that significantly looking ahead, and we are committing ourselves to more than 100x improvement in training time over the next three years.”
During the webinar, a listener asked when deep learning will be able to tackle “real world” problems. Dubey brought up farming as a potential application for deep learning. The problem is even when one narrows the window down to a single growing season for a single field, there are still trillions of parameters to be processed. And that takes an amount of computing power that’s unobtainable—at least for now.
Dubey adds: “Once we get to the point where a trillion-parameter network can be trained in sub-hour—let’s say 20 minutes, 10 minutes—we will have graduated to be able to handle real-world problems with AI. Within three years, we expect to be in that ballpark.”