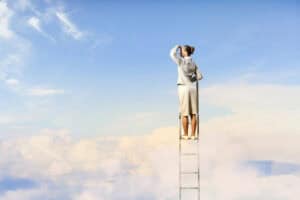
Industry leaders share their insights into the future of GenAI applications. Here is a look at some of the opportunities they find that organizations are investing in now.
RTInsights attended the Gartner Data & Analytics Summit 2024 to hear about the most recent research the firm had done, the insights and recommendations it had distilled from hundreds of inquiries with the companies across industries and technology maturity levels, and both adoption and innovation trends.
We also had an investigative agenda–find examples of real-time data serving to advance AI, especially GenAI use cases, and take the pulse of the rate of adoption, especially given the many discussions around the risk and cost involved. Were companies actually implementing GenAI solutions? And what were they using them for?
Interviewing industry-leading software providers about what they were seeing their customers do resulted in an interesting aggregation of use cases–interesting especially because these software providers viewed the AI and GenAI use cases through the lens of their products’ capabilities and the business problem they focus on solving. These different perspectives result in a composite view of the opportunities organizations see in AI, the ones they are investing in first, and the technology strategies they rely on.
While we focused the interviews on the actual, the present, conversations with the industry’s leading innovators strayed into what use cases were emerging among early adopters and what solutions were still over the horizon but already being conceptualized by these software providers.
A peek into our findings: yes, real-time data processing and analytics are integral parts of some of today’s AI solutions and are essential to many of the solutions in the proof of concept or piloting phase, that is, the final phases on the path to deployment.
See also: Gartner Data & Analytics Summit Delivers on GenAI
Extremely fast data ingestion
Madhukar Kumar, CMO of SingleStore, described his customers’ AI and GenAI use cases that rely on having extremely fast data ingestion to support real-time analytics. At least, that’s the starting point. Surge pricing, fraud detection, and investments management involve millions, sometimes billions, of transactions that must be analyzed in real time. LLMs and Transformers allow AI to be part of those analytics so that users can conduct semantic searches against structured and unstructured data through the addition of a vector database, as requested by an innovative customer several years ago. Now, customers are eager to do meaning-based searches that require finding data clusters, using algorithms to find next-nearest neighbors, and working with contextual data facilitated by retrieval-augmented systems (RAGs).
Beyond this new breadth of search capabilities, customers’ use cases demand extremely fast results. As Madhukar described, SingleStore responded by adding new algorithms: hierarchical, small habitable world, product quantization, and inverted index. Using all of these, the data is compressed by 60 or 70%, and the search can be run in memory, so the search is 200 times faster. Customers can now search across both unstructured and structured data in a few milliseconds and in one SQL statement, so many real-time AI use cases have now become a reality. For example, live video or live conversations can be vectorized in real time and then handed over to an LLM. Financial services, supply chain, ride-sharing, and ad tech have the most appetite for this scenario.
SingleStore does not specifically see GenAI applications deployed yet. Customers are building two types of applications: information synthesis and agentic. They can work together, for example, is a customer agent (human) uses a chatbot that curates all the data and helps the agent answer a question. Agentic takes it further: Based on the curated data, the chatbot can create, close, or update a ticket. Several customers are in the process of building this kind of application.
What’s next? Combining different agentic applications or, more accurately, a mixture of different expert models that work in coordination.
See also: Smart Talk Episode 2: The Rise of GenAI Applications with Data-in-Motion
Unifying analytics and AI for a 360-view
Customer 360 and customer experience use cases still reign as the primary objective of enterprise data initiatives. However, the goal of having a comprehensive view of a customer, let alone a customer base, is still beyond our reach. The more the technology for this use case develops, the more data and analytics methods we uncover, AI and GenAI being the most recent advances that will help us fill in the gaps.
Nik Acheson, Dremio’s Field CTO, identifies supply-chain analytics as another significant area where companies are investing heavily to bring together disparate types of data, historical and real-time, structured and unstructured, to build a holistic view of a complex process that can predict events and recommend options based on current inventory or suppliers’ logistics. What underpins these use cases is query acceleration and unified analytics. The latter is defined as a comprehensive view of data offered by a semantic layer over a data lakehouse that gives users and applications access to clearly identifiable and well-governed data.
Regeneron, a pharmaceutical company, adopted Dremio’s unified analytics, which changed the way research and pre-manufacturing teams interacted with data. One immediate outcome was to save money and align on standardized data when the company realized they were purchasing the same third-party data sets multiple times and stopped the practice. Overall, having a consistent view of, and accelerated access to, their data has sped up their innovation cycles as they can bring products to market faster, continue to test them, and more quickly make improvements based on analyzing previous product introductions.
AI’s Impact on Object Storage
From the perspective of the bottom layer of the data technology stack, AI has been affecting decisions around file systems and object stores from the start, first in research and academics, subsequently in enterprises and clouds, and now in smaller organizations. Ugur Tigli, CTO of MinIO, an object storage provider, has experienced these changes since his days working on financial services data and later on Hadoop. He sees a continuous evolution in AI from statistics to algorithmic trading and then predictive analytics using ML and ever-more advanced models such as LLMs. Ugur explains that “ChatGPT was an inflection point because it allowed the generative AI to become more…mainstream and user-friendly.”
However, ChatGPT adoption is still in the proof-of-concept and piloting stages, at least as far as the demand for more object storage indicates. What enterprises are focused on is bolstering the foundation of their data systems, driven by an appetite to better utilize their data for AI applications like predictive analytics. Problems with architecture, governance, security, and latency have proven to be stumbling blocks for AI, and as Gartner repeatedly highlighted at the Data & Analytics Summit 2024, a flawed data foundation will be an obstacle to enterprise ChatGPT.
See also: Gartner Keynote: Be AI-ready to Empower Change
The most common scenario MinIO finds among its customers is the large enterprise that looks to use AI across its data that must remain private which it equates with keeping the data on-prem. These companies cut across industries but generally keep their data on-prem because of cost concerns and regulatory reasons. Many are located in Europe, the Middle East, and other countries that required data stay within national borders.
The effects of GenAI are already being seen as companies increase their storage infrastructure to accommodate training LLMs on their own data. Another driver for increasing storage is the addition of vector databases or vector capabilities in traditional databases. Vectorization is part of the process for converting or indexing unstructured data like PDFs, images, and video to speed retrieval and allow the use of more complex search algorithms. Both of these use cases are still emerging, according to Ugur, but the early signs are there, namely the growing number of exascale storage footprints.
Data Observability with and for AI
Are companies considering data observability practices as part of getting their foundation ready for AI? It seems that there are too many companies that think they can skip maturing some aspects of the data lifecycle, commonly data governance. Early adopters of GenAI, especially those committed to trusted or ethical AI, warn against this.
Sanjay Agrawal, Co-founder and CTO of Revefi, shared his experience with a new kind of data observability called for as data scales and AI informs more critical decisions. Typically, data observability is adopted to cut costs and improve data quality. These remain important, but automation is now required to manage the vast volumes of data and array of data sources that serve AI applications.
The use of AI to provide context around alerts and events captured by observability platforms is showing data teams a new way to do root-cause analysis and propose very different solutions from what teams have been used to.
Take the problem of a query that uses ⅓ of compute resources. A pre-AI approach could have been to start investigating resource allocation or server behavior itself before looking closely at the query. Once the errant query is identified, you could simply kill it or block it. However, when you take a look at contextual data, you can see that it’s one individual who frequently runs this query. You can even ask ChatGPT or a co-pilot to look at the query for problems, and a problem with the use of locking is identified. Now, the human-in-the-loop steps in and decides how to handle a programmer who needs to adjust their syntax.
A data quality issue can follow a similar path. The first reaction is to find and inspect the relevant pipeline and fix whatever bugs there might be. With contextual data that covers the lineage, the frequency and circumstances of use, you might discover that this pipeline has been abandoned–has no technical or business owner. Sanjay recommends that the best way to address this problem is to eliminate the pipeline after a human-in-the-loop reviews the case.
Enterprise data already exceeds the ability of a handful of experts to oversee it, let alone understand it enough to proactively manage it and take full advantage of it. AI and GenAI will only exacerbate this situation.
The GenAI ecosystem is growing
One sign that a technology is positioned to be broadly adopted is the appearance of software providers focused on supporting development, improving performance, and ensuring it meets enterprise standards. The ecosystem around LLMs has grown rapidly, with established companies adding capabilities to simplify and enhance the adoption of LLMs, especially GenAI applications like ChatGPT.
Alon Gubkin, CTO at Aporia, saw many companies immediately understanding the possibilities offered by ChatGPT. One application dominated: Making company information locked in PDFs or other unstructured objects retrievable so that employees could improve their productivity or better serve customers. Early on a problem with accuracy was detected, namely hallucinations.
Alon reminds us that all models hallucinate–we just used to call it “drift” when structured data was involved. Data scientists have always dealt with drift, but now so many people know stories of odd or damaging hallucinations that the issue is magnified.
One way to address it is to use retrieval augmented generation (RAG) and add context data to enhance a chatbot’s output. More and more companies are doing this, however, at the data level, not per chatbot or application. Often, data engineers build a gateway between applications and data where security checks and data enrichment happen. This check has to happen extremely fast–in real-time using data streams or other approaches such as minimizing the size of LLMs. For example, you can reduce a general-purpose LLM from 300 billion parameters by including only the parameters that matter to your application. Of course, the LLM is “less smart,” which you address by fine-tuning the model for specific tasks. The result of these steps is faster responses with a higher degree of confidence in accuracy on the part of the user.
See Also: Smart Talk Episode 3: Modern Data Pipelines and LLMs
How to differentiate GenAI solutions
“GenAI is a little bit becoming the hammer, and every use case is starting to look like the nail,” observes Jake Bengston, Cloudera’s Technical Evangelism Director. There is no denying that the interest in GenAI is huge, but enterprise adoption is measured because there are many questions to answer about ROI, managing risk, and how to shore up data infrastructure and architecture to take the additional load.
Approaches to resolving these issues are starting to emerge. One barrier is the concern about using third-party LLMs and exposing private data to them. Building your own LLM and training it is just too expensive. Jake reports that many companies are looking at open-source models as an alternative, but they are also looking for ways to stand out when everyone is using the same models trained on generic data.
Jake has seen companies take these approaches to differentiating a company’s GenAI implementation:
- Research models and experiment to find the one that best fits your objectives, testing them on your anonymized or synthesized data and comparing results.
- Reduce the number of parameters and achieve a level of customization by fine-tuning the model to the business case.
- Use RAG to provide context derived from a company’s private data.
- Ensure data architecture and infrastructure are optimized for performance.
Enterprises are succeeding at moving beyond “generic” GenAI, as this example of a company identifying multiple opportunities for GenAI benefits shows. Two key points are that this strategy is being implemented in phases and it is multi-pronged. One of the phases involved creating a co-pilot trained on in-house standards for their developers, resulting in 20% shorter development times. Another was vectorizing unstructured data such as PDFs, PPTs, and graphics to let employees tap into a vast amount of information more quickly through summaries and searching. This has reduced the amount of time spent on research by 95%. Additionally, they use a GenAI chatbot enhanced with the next-best recommendations. Note that the company still uses its existing ML-based applications, using GenAI only when it can prove its value.
See also: 10 Executives on Why and How GenAI Is Here to Stay
Concluding Observations
One of the accelerators to GenAI adoption is the availability of LLMs trained on vast amounts of data. These LLMs, such as GPT, can be deployed relatively easily and have tempted many teams to race through experimentation and pilots, only to be confounded by some of the issues around hallucination and the hilarity and damage it can cause.
Getting beyond the readily available foundations of GenAI to differentiate from the competition is an expensive process due to compute and object storage capacity, data science expertise, and the risk of data privacy and compliance breaches. There are, however, some road-tested approaches to customizing an enterprise GenAI experience. The ROI, however, still needs to be proven. The fact that GenAI affects KPIs is becoming clear, but the cost justification is not, at least not yet.
One of the most important takeaways is that successful GenAI journeys begin with a robust data foundation and progress through business justification (that can include soft values like the importance of being perceived as a modern, agile business), experimentation, differentiation, and phased rollouts. A good indicator of success is previous experience deploying impactful AI and not just doing AI for AI’s sake.
Author’s note: Thank you to the knowledgeable technologists who shared their insights for this article.