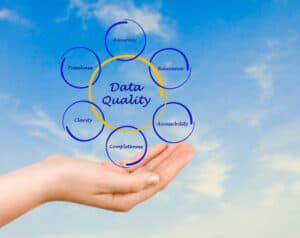
More business leaders and technologists are focusing on improving the data quality behind AI projects to promote more inclusive datasets to take bias out of AI results.
Artificial intelligence and machine learning can deliver amazing insights out of the reach of human analysts, and do so in subseconds. However, the trustworthiness of the insights delivered may be questionable – and even do harm to individuals and companies. That’s because AI’s “intelligence” is limited by the data which it ingests. A bare majority of executives acknowledge this, and few are actually taking active measures to ensure the viability and validity of the data that is fed into their AI models. Hence, the importance of data quality.
That’s the word from Appen, which, in conjunction with The Harris Poll, released the results of a survey of 504 IT executives, which finds 51% of participants agree that data accuracy is critical to their AI use case. To successfully build AI models, organizations need accurate and high-quality data. There is a significant gap in ideal versus reality in achieving data accuracy. “The problem is, many are facing the challenges of trying to build great AI with poor datasets, and it’s creating a significant roadblock to reaching their goals,” the survey’s authors state.
The majority (88%) feel their organization has the necessary internal resources in place to manage data across each stage of AI development – from sourcing to training. However, 42% of technologists find the data-sourcing stage of the AI lifecycle “very challenging.” Business leaders aren’t quite as concerned about the data sourcing challenge – only 24% see this as an issue. “This shows there are still gaps between technologists and business leaders when understanding the greatest bottlenecks in implementing data for the AI lifecycle,” the authors state. “This results in misalignment in priorities and budget within the organization.”
See also: Data Engineers Spend Two Days Per Week Fixing Bad Data
There’s an urgency to achieving greater quality in data being fed into AI systems. More business leaders and technologists are focusing on improving the data quality behind AI projects in order to promote more inclusive datasets to take bias out of AI results. In fact, 80% of respondents stated data diversity is extremely important or very important, and 95% agree that synthetic data will be a key player when it comes to creating inclusive datasets.
The survey covered five key stages of AI data management:
Quality: “Business leaders and technologists report a gap in the ideal versus the reality of data accuracy. More than half of respondents say data accuracy is critical to the success of AI, but only 6% reported achieving data accuracy higher than 90%.
Evaluation: Maintaining fair and accurate AI requires constant attention to the models being trained with the latest incoming data. At least 90% are retraining their models more than quarterly, the survey finds. “AI will not be replacing humans any time soon,” the survey’s authors state. There’s a strong consensus around the importance of human-in-the-loop machine learning with 81% stating it’s very or extremely important and 97% reporting human-in-the-loop evaluation is important for accurate model performance.
Adoption: Uncertainty reigns as to whether businesses are catching up with AI. Business leaders are split down the middle on whether their organization is ahead of (49%) or even with (49%) others in their industry. Technologists are equally split on whether their organization is ahead or even with others in their industry.
Ethics: Responsible AI is the foundation of all AI projects. 93% of respondents agree that responsible AI is a foundation for all AI projects within their organization.
“As a data optimist, I believe the data revolution has the potential to bring immeasurable benefits to people in ways that are only beginning to become apparent,” says Erik Vogt, vice president of enterprise solutions at Appen. “But with this emerging power, comes the potential for harm from abuse or misuse of data, often carelessly or unintentionally. At its core I feel that data ethics is fundamental to our core sense of trust and integrity in, and for, ourselves, as well as in the technology we interact with.”