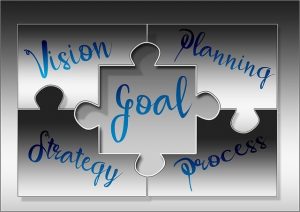
Process mining offers great efficiency improvement opportunities, but proper use must address privacy concerns and protect people’s data privacy rights.
Process mining is a nascent but accelerating market with toolsets used to discover, monitor, and improve processes by extracting knowledge from event logs readily available in today’s information systems. Based on this market definition, it may not be self-evident to CIOs as to how process mining may expose and compromise personal information. The natural inference is that process mining is about uncovering how processes actually behave (versus assumptions), uncover process bottlenecks, and identify areas for process optimization. On the surface, process mining does not appear to have privacy implications.
See also: How Differential Privacy Can Make Your AI Models More Responsible
However, business processes are often case-based, such as employee and customer onboarding, insurance claims processing, and myriad customer service operations. These processes are inherently human-centered in nature, with a high degree of variability in process paths and execution. In this context, privacy implications can arise along two dimensions:
- Process mining of event logs that include personally identifiable employee information gathered during the course of their employment
- Process mining of specific categories of use cases, such as patient journey mapping, loan origination, and call center operations, which may contain attributes associated with third-party personal data.
These process mining instances highlight privacy challenges and the need to implement organizational and technological measures that demonstrate the protection of data subject rights, which include adherence to privacy by design principles. Privacy by design encompasses seven key principles; perhaps the most important is the principle of privacy as a default setting. It means that the collection of personally identifiable information ought to be pursuant to a lawful basis for processing, limited to what is absolutely necessary and processed consistent with the purposes for which such information was initially collected.
Where Privacy Considerations Impact Process Mining Outcomes
The starting point for process mining is an event log. Event logs store information such as the resource (person or device) executing or initiating an activity, an event’s time stamp, or data elements recorded with an event (such as the size of an order). Organizations can use event logs to “improve processes based on facts rather than fiction.”
Event logs may contain direct and indirect identifiers of personal data, and disclose personally identifiable information, for example:
- Analysis of customer-facing processes such as insurance claims, loan origination, and customer call centers may include event logs that reveal how exactly how work is getting done across automated activities and human workflow steps
- Compliance driven processes such as data breach readiness, Know Your Customer, and Fraud Detection may contain sensitive event log data
- Patient journey mapping and health care delivery centered process analysis includes protected health event logs
- IoT devices are becoming pervasive in the workplace. They collect enormous volumes of information in real-time, which may form part of an event log. And, invariably, they may indirectly reveal personally identifiable information such as location, habits, performance, and physiological attributes. These attributes are defined as quasi-identifiers, which means that in isolation, they may not identify a data subject, but in combination with other data sources, they may.
Use Deidentification Methods to Safeguard Privacy
Deidentification of personally identifiable information is a well-established methodology for safeguarding privacy rights. There are two generally accepted techniques associated with deidentification: anonymization and pseudonymization.
Anonymization provides the most stringent mechanism which permanently removes any direct identifiers of personal information, but impacts the utility of process mining results.
Pseudonymization means that processing of personal data cannot be attributed to data subjects without the use of additional information. However, it may still be possible to reidentify personal information from pseudonymized event activities through brute force attack or by an adversary who may be familiar with the data set.
Generalization is another possible method of deidentification whereby specific attributes within event logs may be aggregated to a more general or broader value. However, by doing so, the utility of process mining to identify variances and outliers in process execution may be adversely impacted.
Best Practices to Ensure Privacy with Process Mining Tools
There are a few ways CIOs can ensure deidentification methods are successful while maintaining the integrity of process mining benefits.
1) Assess the risk of reidentification associated with analysis of event data. Clearly, if the event data contains personally identifiable or sensitive personal information, it must be anonymized and substituted with a replacement value. However, there still may be a possibility of reidentification based on combining event log attributes with other available data sources.
2) Mitigate the possibility of reidentification with a data governance structure and policy. Four guidelines to consider:
- Evaluate the intended uses and users of event logs collected for analysis
- Determine the variables included in event logs
- Measure their reidentification risk
- Document results consistent with data privacy and security requirements.
3) Control the terms when data is used. There are different “release” models associated with the secondary use of personal information: public, quasi-public, and non-public release models. Public release models should apply the most stringent de-identification protocols while quasi and non-public release models ought to include specific contractual provisions as to the confidentiality and terms of use.
4) Evaluate the nature of the variables in event logs by asking a few questions: Do they contain sensitive data? Do they include indirect identifiers that may create risk of reidentification? Are there additional sources of publicly available data sources that may be linked to indirect identifiers in event logs? What is the likelihood that an adversary who may be familiar with the event logs and be able to reidentify data subjects?
5) Measure and identify reidentification risk. That will depend on the context of event logs, the number of attributes that comprise event logs, and the number of similar attributes, referred to as equivalence classes. The fewer the equivalence classes, the higher the degree of probability of reidentification. In such instances, more rigorous deidentification measures ought to be considered.
6) Document internal controls that protect privacy rights. The General Data Protection Regulation imposes rigorous obligations on data controllers and processors to maintain a record of processing activities under its responsibility. Furthermore, organizations are subject to audit provisions and, upon request from supervisory authorities, must cooperate with the supervisory authority and make those records available.
Process Mining and Data Privacy can Co-exist
Process mining can provide your organization with comprehensive insights about your processes and fuel your improvement initiatives. The ambition of responsible process mining is to achieve a balance between its utility and safeguarding privacy rights. Integrating privacy-enhancing technologies and best practices will engender trust and confidence in their continued growth. It is in the best interest of industry and vendor communities to espouse privacy enhancing practices.