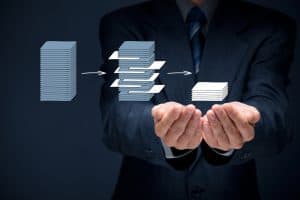
Taking the time to articulate the value data accuracy and agility might be just the thing you need to transform your data preparation efforts and educate stakeholders.
When building a business case for data prep, it’s important to carefully scope and define how the solution can help. A good way to start is by looking at the following benefits and asking yourself how clean and trusted data, at an accelerated rate, can be applied to generate a competitive advantage:
- Data accuracy: Can you claim a bigger slice of your industry’s market share or avoid large, significant costs because of more accurate data?
- Data agility: Can you reach new revenue or save costs because you have access to the right information faster?
See also: Building the Data Prep Business Case Part 1: Five Scenarios to Quantify ROI
Measuring the Total Value and Assigning an Improvement Range
To measure the ROI of data preparation, we will breakdown the measurement into two areas.
Total Value (TV): this is the total improvement achieved by having better data quality or data agility. This improvement can depend on several factors, including but not limited to, data preparation tools and solutions that improve the quality and agility of data. For example, the experience of the team, the skills they possess, and other related technology solutions (e.g., data enrichment services or business intelligence tools) all contribute towards improving the quality or agility of data.
Improvement Range (IR): Once you have defined the TV, the next step is to assign an Improvement Range (IR) that a data prep solution or tool can provide towards the TV. IR will indicate the portion of the TV that is directly tied to the data preparation technology.
For example, imagine you are an insurance company, and the rate in which you set your premiums is directly related to the perceived risk of insuring. However, you may be missing data points such as the accurate income of a household, the age or job classification of an insured, or you don’t have accurate data on the amount of driving they do per year. With more accurate and complete data, you have a more accurate lens to set your premium policies.
All too often, organizations are manually preparing data. They are forced to rely on data science teams to collect, clean, and complete data from different sources using code before feeding it into risk assessment and predictive models. Unfortunately, this approach is not 100 percent reliable resulting in data quality issues that can have a direct impact on your premium rates and your revenue.
To build a business case using the above example, first measure the total value (TV) and then take a portion of that and attribute it to clean and accurate data that is generated from a data preparation solution using the following steps:
- Total Value (TV): Missing data values and lack of completeness cost an average of $X per premium per year. With over Y premiums at play, this will cost an average of $X*Y per year. Understanding that some of this is unavoidable and it’s the nature of the business, assume that Z percent (e.g., 20 percent) can be addressed with higher quality of data. In this case $TV = $X * Y * Z percent.
- Improvement Range (IR): Using a data preparation solution – one that is automated and intelligently cleans the data – will help you not to miss any values and arrive at more accurate information. Assign a range to this improvement, for example, anywhere between IR1 percent to IR2 percent (e.g., 30 percent – 40 percent) improvement.
Then calculate your ROI. In this case. Data Prep Business Value would range anywhere from $TV * IR1 percent to $TV * IR2 percent.
TIP: Never determine an absolute number. Leave your IR as a range with an upper and lower scale for the best case vs. worst case scenario. This will help your executives justify the expenses and will also give you some wiggle room. As you onboard and start using your data preparation solution, you should also measure actuals vs. the target range.
Now let’s imagine you are an online retailer. You receive new merchandise every day, and the quicker you onboard the new product information into your online catalog, the sooner you can reach revenue. However, different manufacturers are sending you data in a variety of formats, and some of the newer products may not fit into your defined categories. You may also need to onboard new suppliers that require you to intake and validate their datasets. In either case, these can be a very manual and lengthy process.
To build a business case for data preparation in this scenario, you first need to first think about the Total Value (TV) that you can achieve by having a more accelerated data intake and higher quality data:
Total Value (TV): With faster onboarding of new incoming product data, we can increase our inventory turnover, making our Days Sales of Inventory (DSI) (i.e., the days it takes for inventory to turn into sales) lower. For example, with quicker data intake, we could go from 60 DSI to 40 DSI, which equates to roughly $10 million of additional revenue annually. Some of the speed and turnover gains may be due to better marketing and promotions, and some may be coming from more accurate predictions of the demand. With this in mind, let’s only attribute 30 percent of your DSI to better data quality and data agility. That makes the Total Value that you attribute to better and faster data: $TV = $10M * 30 percent= $3M.
Improvement Range (IR): Not all the TV can be attributed to our data prep tool. You will need to consider the agility of your data team and/or the fact that you may get standard data input from vendors that do not require heavy re-formatting and cleaning. Let’s assume that only 30 to 40 percent of your data preparation agility depends on the data prep solution, that equates to a $3M * (30 percent) to $3M * (40 percent) = $900,000 to $1,200,000 value.
Buttoning Up the Business Case—Don’t Forget the TCO
When creating the business case, don’t forget to consider the Cost of Ownership (TCO) and how it can vary depending on the solution. For instance, some legacy data preparation tools are heavier in the upfront license costs and lower on the perpetual yearly maintenance costs. This type also tends to require hefty implementation costs and significant change management. Alternatively, SaaS models have a yearly subscription fee, but if you deploy your SaaS on-premises, you also need to consider hardware, hosting, and manual upgrade costs.
It’s also important to calculate current data preparation costs if your organization is considering adopting data prep solutions because they want to re-vamp old processes. These can include removing the company’s reliance on manual coding, eliminating the use of Excel spreadsheets that are unable to scale, or replacing legacy ETL solutions that are resource-heavy.
As you put the finishing touches on building your business case, make sure to project the costs for the next 3 to 5 years, and consider all cost drivers as well. These can include change management and service costs as you bring new data sources in or increase the footprint of your data prep to cover additional use cases.
TIP: It’s always better to project a best-case and worst-case scenario and articulate the minimum requirement that it takes to break even. Also, be sure to review your assumptions and improvement projections with your sponsors and stakeholders along the way, so by the time the business case is final, nobody will be seeing it for the first time.
Lastly, remember to focus on the qualitative storyline as much as the numbers. After all, it’s always better to have a viable use case or example of improvement to gain credibility for how the new solution could make an impact.
As data continues to become more complex and the agility to get to data-driven decisions more important, the old methods of ensuring data accuracy are proving to be ineffective. This has created a tremendous amount of interest in redesigning old data integration and data preparation processes as organizations look to embrace modern self-service data preparation techniques. Taking the time to articulate the value data accuracy and agility has to the business might be just the thing you need to transform your data preparation efforts and educate stakeholders on why they need to adopt this progressive approach.