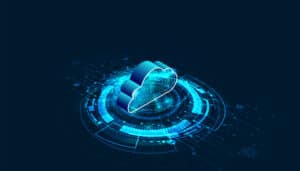
The huge rise in data from various sources is forcing observability practitioners to modify their approaches considerably.
Can there ever be too much data in big data? The answer is a resounding yes – and this is increasingly true when it comes to modern observability initiatives – or the task of measuring the current health of apps and systems based on the external data (logs, metrics, and traces) being generated.
In fact, today’s organizations with observability practices in place are dealing with such a huge data influx that they can no longer harness and leverage this data to its fullest potential. Over the past decade, enterprise data volumes have scaled from gigabytes to terabytes to petabytes, and nearly all IT practitioners believe that current tools and approaches aren’t sufficient for solving business problems.
What’s driving this data surge? It’s everything from the rise in cloud adoption and hybrid infrastructures to microservices and continuous delivery models – all of which are increasing the sheer volume of production environments (and consequently, data sets being generated) that organizations have to manage. For many, this is a case of big data simply getting too big – quickly reaching a point of diminishing returns. It generally takes longer to find and fix poorly performing services that ultimately can impact the customer experience and the bottom line.
See also: 8 Observability Best Practices Every Org Should Implement
All of this is forcing observability practitioners to modify their approaches considerably. More specifically, they are moving away from working with huge masses of unstructured information (big data) to consolidating this data into chunks (manageable data), all processing in parallel. These practitioners are:
Doing Away with ‘Centralize then Analyze’ As an Approach
Observability practices have traditionally been based on a ‘centralize then analyze’ or ‘store and explore’ approach. This means all data is ingested into a centralized monitoring platform before users can query or analyze it. The rationale behind this approach is the more data you have, the more you can correlate, and the richer the data becomes contextually.
There are two main problems with this approach. First, it is becoming far too costly. Many organizations can no longer afford to store all their data in hot, searchable storage tiers. Second, this approach leads to slowness – both in terms of the time the centralization process takes, as well as creating overstuffed central repositories, which require much longer wait times for data queries. Even a few extra minutes of delay in getting an alert can make a big difference in mission-critical environments.
To avoid these problems, many organizations resort to randomly omitting certain datasets. But what if a problem occurs and this omitted data is precisely the data that’s needed for troubleshooting? You now have two choices: (1) take the risk and omit certain datasets, or (2) route everything downstream, overwhelm the platform, and pay full price (even if you only use a small fraction of the data indexed).
Analyzing data as it’s being generated at the source: Clearly, ‘centralize then analyze’ is no longer a viable approach. A new method reverses this paradigm by applying distributed stream processing and machine learning at the data source so all individual datasets can be viewed and analyzed in parallel as they’re being created.
When we move from big data to manageable data in this way, there are several benefits. First, organizations always have full access to all the data they need to verify performance and health, as well as make necessary fixes whenever a problem is detected. Painful trade-offs between cost overages and having complete access to data (invaluable for peace of mind) no longer have to be made. Once it’s pre-processed, normal/nonessential data can be routed to inexpensive cold storage for safekeeping, helping reallocate your index license to only data you actively use.
Second, when you pre-process data as it’s being created at the source, you are alerted on every issue as soon as it occurs and shown its exact location, which is critical in modern production environments where workloads are constantly proliferating and shifting. Without this capability, it becomes too difficult and time-consuming for organizations to sift through rapidly growing piles of log data in order to correlate an alert to a root cause.
Doing More with Less
Another benefit of analyzing data at the source is that organizations become much more nimble in conducting real-time data analytics. This helps solve business problems by identifying growing hotspots and their root causes faster, which is critical for reducing MTTR.
Some organizations have so much success processing data at the source that they find they don’t even need a central repository at all. But for those who wish to keep one, high-volume, noisy datasets can be converted into lightweight KPIs that are baselined over time, making it much easier to tell when something is abnormal or anomalous. In this way, organizations can “slim down” their central repositories and maintain their speed by better policing what’s getting sent there.
Making More Data Accessible
The reality is there are going to be times when access to all of this data is needed, and it should be accessible. While having all of one’s data in one central place would seem to have a democratizing effect (it’s all in one place where everyone can access it), it’s often the opposite in complex, big data environments, where developers rely on experts to maintain application health and performance. Condensing repetitive data into patterns, regardless of the storage tier they’re in, can be the key to simplifying the monitoring and troubleshooting process for developers. This approach helps developers work independently and solve problems on their own – which is important as more organizations emphasize having developers “own” their applications.
In conclusion, data is the world’s most valuable commodity, and it’s natural to think that the more data you index, the better. But there are situations where organized data beats big data, and modern observability initiatives prove to be essential. as ever-expanding data volumes become overwhelming for humans to review or grasp. Ironically, moving from downstream- to upstream is not swimming against the current but rather an evolution – enabling more agility in reaching important conclusions faster, more reliably, and at a lower cost.