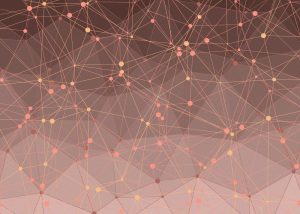
The logical data fabric is a vision of a unified data delivery platform that abstracts access to multiple data systems by hiding complexity and exposing data in business-friendly formats.
In today’s data-first economy, it is not unusual for some organizations to have multiple data science, data engineering, and data platform teams dealing with issues such as pricing, supply-chain, and in-store shopping-related advanced data analytics and data science to propel their business and achieve a competitive advantage. As a result, one of the biggest challenges IT teams face these days is to serve a broad range of data consumers with varying levels of skill-sets. That is why a logical data fabric approach is rising to prominence.
Such an approach promises to achieve a flexible, real-time, and augmented data integration pipeline, combined with comprehensive data management capabilities, to serve the most data-savvy as well as the least data-savvy consumers within an organization. By utilizing knowledge graphs, data catalogs, and AI/ML on active metadata, this new data integration, and data management approach supports faster and automated data access and sharing.

A data fabric is a composable architecture, meaning components like data catalog, knowledge graph, data preparation layer, recommendation engine, DataOps, and orchestration can be combined with disparate tools working together. While that is true, some of the best-of-breed data fabrics are a single platform, offering all the important capabilities of a data fabric. The logical data fabric is a vision of a unified data delivery platform that abstracts access to multiple data systems for business consumers, hiding the complexity and exposing the data in business-friendly formats while, at the same time, guaranteeing the delivery of data according to predefined semantics and governance rules. In the digital world we live in, it is not much of a stretch to say that will make every CIOs dream come true.
A key criteria of today’s self-service strategy is the ability of business users, such as citizen analysts, data scientists, and LoB developers, to find what datasets are available in the data delivery layer to ascertain which ones are relevant for their information needs. Dependency on the IT team for data search and discovery has become a bottleneck for personas such as data scientists and citizen analysts. Worse, these scarce resources cannot afford to waste wrangling data rather than building models or analyzing data. A data catalog that is integrated with the data delivery layer and is enhanced with an AI/ML-based recommendation engine helps users achieve fast data discovery and exploration. Business stewards can create a catalog of business views based on metadata, classify them according to business categories, and assign them tags for easy access. A logical data fabric with enhanced collaboration features helps all users endorse datasets or register comments or warnings about datasets, helping them to further contextualize dataset usage and better understand how their peers experience them.
While ease of data search, data discovery, data classification, and tagging help users to find the right data at the right time, it can be significantly enhanced with the help of a powerful AI/ML engine. In an AI/ML-powered logical data fabric, past user activity can be analyzed to provide personalized recommendations and shortcuts to select datasets, which accelerates data science projects and advanced analytics. Other enhancements may include extended profiling information about datasets and columns and improvements in smart search, which is the smart ranking of results, similar to the way google search works but in the context of enterprise data access.
As companies spread out their operations across the globe, so does their data. More importantly, enterprise-wide data is not only distributed regionally but also across clouds, sometimes multiple clouds and on-premises. While a logical data fabric architecture holds the promise of non-replication of data to a large extent, that also begs the question of performance of queries run by data scientists, business analysts, or LoB users, a key criteria for making a rapid business decision. A single query may potentially access data from multiple different locations across the globe, with a mix of cloud and on-premises systems. While there are many possible ways of accelerating the query, including caching, query pushdown, etc., one of the most useful query accelerations can be accomplished with the use of AI/ML.
Data consumers, such as data scientists, citizen analysts, or executives, are often looking for information that have related or the same intermediate datasets. In such cases, this intermediate stage information can be intelligently materialized and stored in data repositories that are most optimal for data access at a later time. During a similar query run, the AI/ML engine can recommend the usage of a materialized view to accelerate the query by many fold. It can greatly accelerate business decision-making, which can help increase revenue and/or decrease costs.
Both the query acceleration and data catalog-based enhanced collaboration and data search are made possible with the combination of metadata activation and AI/ML techniques. Metadata truly holds promise for the future of data management and digital business transformation. Comprised of a foundation of metadata-based data integration, data management, and data delivery, the hope is that a well-planned logical data fabric that includes critical capabilities such as data virtualization, an integrated data catalog, and a strong AI/ML-based recommendation engine can solve complex enterprise-wide data access problems and enable organizations to serve their customers better.