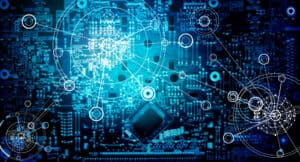
In data-informed organizations, AI/ML and workflow orchestration function as a kind of intelligent research assistant, operating at scale without interruption.
Big data is all the rage. Everyone seems to understand the whole “data is new oil” paradigm, but most organizations are still struggling with the tasks of extracting, refining, and ultimately delivering value with all those newfound riches.
The majority are inclined to steer their organizations toward a so-called “data-driven” approach to decision-making. Given the significant investments that companies are making in advanced analytics and AI/ML, it’s well worth examining that phrase in more detail. What exactly do “data-driven” decisions look like?
Conventional wisdom provides us with two answers to that question. The first is an executive-level BI approach to decision-making that we might call strategic analytics. This is about exploring the data, asking new questions, and discovering insights that can help to shape the strategic direction of the organization.
The second category of data-driven decisions is about automating routine operations using AI. Paired with strictly defined rules-based workflows, this diminishes the need for human judgment to enter into the decision-making process. It enables a shift toward management by exception. This paradigm is often supported by the notion that machines are better at making decisions than humans. In certain scenarios, that may be true, but it’s simply not valid as a blanket proposition.
There is a third approach to creating business value that most people seem to be overlooking. It’s not about free-form exploration in the C-suite, nor is it about eliminating the human element from operational decisions. The third approach is a “data-informed” business process.
Unlike a data-driven model, the data-informed approach ensures that human beings remain firmly planted in the driver’s seat. At the same time, it offers a proven, structured framework for turning big data into measurable business value. Let’s explore what this looks like in the insurance industry.
See also: Where Is Our Data? Why Data Location Discovery Is Hard
What does it mean to be “data-informed”?
Complex, nuanced domains like casualty insurance claims can benefit greatly from advanced analytics, but they’re poorly suited to the kind of blind operational automation that removes human judgment from the picture altogether. Claims typically involve thousands of different variables. They require careful reading and a deep contextual analysis based on medical records, accident reports, and other documents. Claims managers must look for subtle details that suggest potential risks or opportunities. In short, human experience matters.
Can algorithms achieve the same outcomes? To some extent, yes. But in highly complex domains like workers’ compensation claims management, there is simply no replacement for having an experienced claims professional with eyes on the details of each case. Advanced data analytics can give that person a substantial advantage by informing and empowering them to make the best possible decisions. That’s the essence of a data-informed approach.
In data-informed organizations, AI/ML and workflow orchestration function as a kind of intelligent research assistant, operating at scale without interruption. The technology is there to flag potential risks, highlight anomalies, and monitor for situations that call for immediate attention. In this context, AI doesn’t replace human judgment; it optimizes the application of human judgment by providing a very meaningful assist.
The case for a data-informed approach
In the commercial insurance space, the technology marketplace has focused largely on data-driven applications. The implication is that claims agents should be dialing back the human factor and making decisions solely based on what the analytics are telling them. That’s a flawed approach, though. After all, you can build business rules, but it’s impossible to capture every possible nuance that might come up in the course of a claim.
That leaves adjusters to make a personal risk-reward calculation. They can spend their time and energy trying to understand each individual case and render the best possible decision, or they can default to a rules-based approach and fast track everything that comes across their desk. The former approach is dependent on hard work and good judgment, but the latter is low effort and low risk. Naturally, the vast majority of claims adjusters will simply opt to follow the data-driven conclusions that the system hands them.
A data-informed approach, in contrast, places full ownership of the decision-making process on claims managers, but it adds a depth of perspective that simply isn’t available using conventional methods.
Laurie Pierman, VP of Claim Operations and Shared Services at Amerisure, offered a vivid example at the last National Comp conference. A group of Amerisure’s experienced claims managers were frequently referring cases to a specific doctor. Many had long-standing relationships with the physician, sometimes dating back to their work at previous carriers.
When Amerisure rolled out a new claims intelligence solution, those adjusters were surprised to see that this doctor had been scored lower than expected by the AI engine. Digging deeper, they discovered a problem: unusually high costs driven by an alarming volume of opioid prescriptions.
Although some of those adjusters had worked with this doctor for 15 years or longer, no one had ever put these pieces together before. AI discovered the anomaly, and a team of experienced claims professionals put it to practical use. The carrier is saving money. More importantly, they’re steering patients toward physicians who will provide better outcomes for the injured employees they serve.