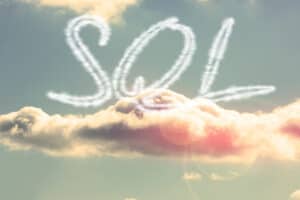
A dizzying range of tools and services has grown out of the need to speed up and scale data analytics. However, one skill that has often been overlooked is humble SQL, the data scientist’s best friend.
Data engineering and data science are fast-moving, competitive fields. Technologies come and go, so keeping your skillset updated is something all ambitious data pros can agree on. Where data engineers and scientists disagree is exactly what skills will be most valuable in the future.
Regardless of the bewildering array of tools and services available to data scientists, it is still humble SQL that forms the bedrock of a data scientist’s stack. While SQL is usually seen as a baseline skill, it is, in fact, much more than that. Despite being almost 50 years old, SQL is becoming more, not less, relevant. Machine learning, big data analytics, or AI may steal the headlines, but if you want to hone a smart, strategic skill that can elevate your career, look no further than SQL. Here’s why.
SQL Dominates Databases
First off, “SQL is really the language of data,” in the words of Benjamin Rogojan (aka Seattle Data Guy). This is down to the fact that the majority of databases are built on one or other of the SQL-based technologies. All but two of the top ten most popular databases today are based on SQL, the exceptions (MongoDB and Redis) are ranked fifth and sixth, respectively, and even they can be used with SQL. It’s easy to see why anyone who needs to query, update, change, or in anyway engage with data in relational databases is going to be well-served with a solid working knowledge of SQL no matter what specialism they end up pursuing.
See also: Python Top Programming Language, But SQL Gets You Noticed
Demand for SQL Skills is High and Growing
Despite its age, SQL is far from a legacy skill. As data engineering has advanced into the cloud, so SQL has followed, and, according to Dataquest, SQL was the most in-demand skill among all jobs in data in 2021, especially at the more junior end of the spectrum. However, even more experienced data scientist job postings still list SQL in almost 60 percent of vacancies.
What’s more, doubtless due to surging demand for data-related expertise, demand for SQL skills appears to be growing, despite a brief dip in 2020. Pandemic aside, the SQL server transformation market – that helps business address the need for data transformation – is predicted to grow steadily at more than 10 percent CAGR until the end of the decade.
Should Savvy Data Scientist Prioritize SQL?
The future of SQL looks safe, but it does not necessarily follow that budding data scientists who already have a working knowledge of it will prioritize deepening their SQL skills to further their career progress. They should.
With so many tools and nascent technologies to help them at the ELT / ETL stage, for BI and for both predictive and historical analytics, data scientists need to be savvy about where to plow their energy. The continually shrinking half-life of high-tech skills means that the tools and skills data scientists learn can be career-defining – or career-limiting.
See also: R and Python: Critical Programming Languages to Begin Your Data Science Career
How SQL is Taking Center Stage
No one wants to spend six months figuring out a tool that only delivers half of what was expected of it, let alone recommending it to the wider team, only to find that it underwhelms. So, when data scientists look at the services and techniques out there that will help them query their data more effectively, they are probably going to look at the best BI tools and ML extensions that will enable them to prep the data, create the model, and then train it. But all these different stages take time and demand high levels of expertise. We’ve been conditioned into accepting that ML modeling requires the data to be extracted from the database, usually using a BI tool, transformed and loaded into the BI system, before being exported (again) to the ML tool, where the magic happens, and transporting it back to the BI tool for visualization.
What if I told you there is a way of taking ML models to the data, enabling you to query predictions from inside the database using – you guessed it – SQL? There is.
It’s part of a small but rapidly growing movement that brings intelligence into the data layer rather than painstakingly taking the data to the ML tool.
In-database Innovation
In-database ML is a much simpler way to use existing data to predict future events…and it uses standard SQL commands. In-database ML is a bit like giving your database a brain. It means data scientists – and data engineers, and indeed anyone with SQL skills – can work within the database, running ML models to answer almost any business question. Predicting customer churn, credit scoring, customer lifecycle optimization, fraud detection, inventory management, price modeling, and predicting patient health outcomes are just a few of the many use cases in-database modeling has enabled. With this approach, all the ML models can be created, queried, and maintained as if they were database tables, using the SQL language and bringing powerful predictive capabilities to a much wider range of data pros.
In-database ML is a relatively new field, but it is one part of a wider, fast-growing movement to simplify and democratize data engineering and data science, breaking down the technical barriers that currently exist for those working with data. Take, for example, dbt Labs, a company that’s taken the data world by storm, having recently secured $222 million of funding and been valued at $4.2 billion. Its data transformation product enables data engineers to build production-grade data pipelines from within the data warehouse using SQL commands, radically simplifying, speeding up, and scaling the process of prepping data.
SQL – Not Old, But Evergreen
We’re fortunate to be living in a golden age of digital innovation. However, against a business backdrop that prizes the insights data can offer, data scientists are under pressure like never before to produce miracles out of data. A dizzying range of tools and services has grown out of the need to speed up and scale data analytics. These tools often demand an investment in time and skill development to fully realize their benefits. However, one skill that has often been overlooked is humble SQL, the data scientist’s best friend. SQL is not only not going anywhere, but as the growing movement to innovate closer to the data shows, SQL is becoming the data scientist’s strategic secret weapon.