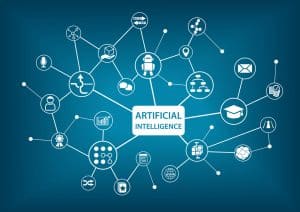
Research shows that an inadequate or lack of purpose-built infrastructure capabilities is often the cause of AI projects failing.
As the use of artificial intelligence (AI) becomes more mainstream and used in more aspects of daily operations, businesses start out by relying on compute infrastructures traditionally employed for HPC. While that approach offers some help, increasingly, what businesses find is that they need an infrastructure that is purpose-built for their AI workloads. That was the findings of a new IDC study.
Specifically, IDC recently debuted its AI InfrastructureView, a deep-dive benchmarking study on infrastructure and infrastructure-as-a-service adoption trends for artificial intelligence and machine learning (AI/ML) use cases. The global survey will be run annually and will include 2,000 IT decision-makers, line of business executives, and IT professionals, the majority of which influence purchasing of AI infrastructure, services, systems, platforms, and technologies.
The survey results show that while AI/ML initiatives are steadily gaining traction, with 31% of respondents saying they now have AI workloads in production, most enterprises are still in an experimentation, evaluation/test, or prototyping phase. Of the 31% with AI in production, only one-third claim to have reached a mature state of adoption wherein the entire organization benefits from an enterprise-wide AI strategy. For organizations investing in AI, improving customer satisfaction, automating decision making, and automating repetitive tasks are the top three stated organization-wide benefits.
“IDC research consistently shows that inadequate or lack of purpose-built infrastructure capabilities are often the cause of AI projects failing,” said Peter Rutten, research vice president and global research lead on Performance Intensive Computing Solutions.
See also: How API Platforms Can Drive Digital Transformations
AI workloads need love
Several key findings emerged from the survey.
AI infrastructure remains one of the most consequential but the least mature of infrastructure decisions that organizations make as part of their future enterprise. Organizations have still not reached a level of maturity in their AI infrastructure – this includes initial investments, realizing the benefits and return on investments, and ensuring that the infrastructure scales to meet the needs of the business. High costs remain the biggest barrier to investments leading many to run their AI projects in shared public cloud environments. Upfront costs are high, leading many to cut corners and thus exacerbate the issue. People, process, and technology remain the three key areas where challenges lie and where organizations must focus their investments for greater opportunities.
Dealing with data is the biggest hurdle for organizations as they invest in AI infrastructure. Businesses lack the time to build, train, and deploy AI models. They say that much of their AI development time is spent just on data preparation alone. Many also lack the expertise or the ability to prepare data. This is leading to a new market for pre-trained AI models. However, like anything off the shelf, pre-trained models have their limitations, which include model availability and adaptability, infrastructure limitations to run the model, and insufficient internal expertise. Model sizes are also growing, making it challenging for them to run on general-purpose infrastructure. Organizations do expect that once they have crossed this hurdle, they will shift their efforts to AI inferencing.
AI infrastructure investments are following familiar patterns in terms of compute and storage technologies on-premises, in the public cloud, and at the edge. Businesses are increasing their investments in public cloud infrastructure services, but for many, on-premises is and will remain the preferred location. Today, for AI training and inferencing, it is divided equally between cloud, on-premises, and edge. However, many businesses are shifting towards AI data pipelines that span between their data center, the cloud, and/or the edge. Edge offers operational continuity where there is no or limited network connectivity.
Security/compliance and cost also play a role. GPU-accelerated compute, host processors with AI-boosting software, and high-density clusters are top requirements for on-premises/edge and cloud-based compute infrastructure for AI training and inferencing. FPGA-accelerated compute, host processors with AI-boosting software or on-prem GPUs, and HPC-like scale-up systems are the top 3 priorities for on-premises/edge-based compute infrastructure for AI inferencing. In the cloud, the highest-ranked priorities are GPU acceleration and a host processor with AI-boost, followed by high-density clusters. More AI workloads use block and/or file than object at this point.
“What is becoming clearer is that gaining consistent, reliable, and compressed time to insights and business outcomes requires investments in purpose-built and right-sized infrastructure,” said Eric Burgener, research vice president, Storage and Converged System Infrastructure at IDC.