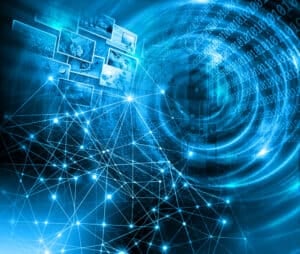
Edge AI offers many benefits making it a powerful tool to enable use cases across a wide range of industry applications.
For years, artificial intelligence (AI) was a cloud-centric proposition. Companies routinely used the cloud’s highly scalable compute capacity to train models, run AI algorithms against massive datasets, and more. But more recently, AI has moved to the edge.
In a way, AI-enabled edge applications are the confluent of a perfect storm of factors. There has been explosive growth in the number of edge devices. And many of those devices are generating orders of magnitude more data than comparable ones from just a few years ago.
For example, in the past, a sensor on a piece of industrial machinery might take one, two, or a half dozen status measurements once a minute or once an hour. Now, there are devices that make dozens or more measurements every second. As a result, the International Data Corporation (IDC) forecasts that there will be 41.6 billion IoT devices in 2025 capable of generating 79.4 zettabytes (ZB) of data.
Another contributing factor in using edge AI is that edge devices now have the compute power to run algorithms and analyze the generated data. In general, edge AI is used for inferencing (in contracts to the model training done in the cloud). To that end, AI inferencing involves putting a model to work on live data to produce insights and actionable output.
See also: Streaming Data Management for the Edge
Industry edge use cases
Edge AI offers several benefits. First, it reduces bandwidth requirements and costs. All generated data does not have to be sent to the cloud for analysis. Second, it reduces latency. When inferencing is performed locally, at the edge, the roundtrip transit time to and from the cloud is eliminated. Third, it can improve reliability. AI can continue to operate even if a network connection between the device and the cloud or a data center goes down.
These benefits make edge AI a powerful tool to enable use cases across a wide range of industry applications. Some examples include:
- IT operations: Using full-time monitoring for a server stack, a large corporation can learn from incidents as they are resolved and troubleshoot potential incidents before they occur. As edge AI is used more for these purposes, those predictor failure alerts become even more critical in identifying problems before they occur. That alleviates users from experiencing any downtime at all.
- Manufacturing: Production lines are increasingly embedded with smart sensors collecting a wealth of data about the status of equipment and processes. In some cases, sensors on each component of the manufacturing process generate more than 1000 characteristics per second. Edge AI can help detect anomalies and send an alert to remedy the situation before it travels too far down the manufacturing line.
- Modern amusement parks: Amusement park operators compete to have the scariest and wildest rides, like rollercoasters. As the rides have become more complex, they are turning to AI-edge insights to keep the rides running smoothly and safely.
- Healthcare: Wearable health monitors are becoming more widely used for many types of ailments. Some smart device manufacturers and healthcare organizations are assessing heart rate, blood pressure, glucose levels, and breathing locally using edge AI models developed in the cloud.
In many cases, the insights derived from AI inferencing at the edge can either be shared with other edge elements or fed back to the cloud to improve the AI model they are based on. In the former case, a smart traffic camera could share derived details to automatically adjust light timings to optimize traffic throughout a city. In the latter case, information gleaned about the performance of a robotic assembly element could be shared with the cloud to look for the root cause of performance anomalies. Any insights derived by analyzing the historic and real-time streaming data from the device could be used to reduce robotic downtime and increase the efficiency of a production line.