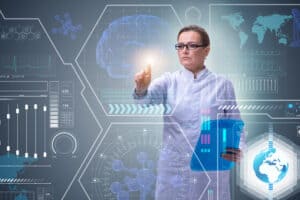
MIT researchers have used a generative AI system which could create novel proteins that are more climate friendly that ones found in nature.
AI researchers at the Massachusetts Institute of Technology have developed a machine learning algorithm capable of generating proteins with specific properties, which surpass what can be found in nature by potentially reducing the carbon footprint in manufacturing and utilizing them.
The algorithm, built by MIT, the MIT-IBM Watson AI Lab, and Tufts University, works in a similar way to the generative AI systems which have taken the internet by storm, such as DALL-E and ChatGPT. But instead of creating images or text from prompts, the algorithm is able to generate proteins structures.
SEE ALSO: Researchers Use AlphaFold To Design Cancer Drug
Researchers created this algorithm by combining two models, one which tackles the protein properties, and the other which works on the amino acids. The first model allows a user to input structural properties, and the amino acid model lets them fine tune the structures to meet specific requirements.
“When you think about designing proteins nature has not discovered yet, it is such a huge design space that you can’t just sort it out with a pencil and paper. You have to figure out the language of life, the way amino acids are encoded by DNA and then come together to form protein structures. Before we had deep learning, we really couldn’t do this,” said Markus Buehler, professor of civil and environmental engineering at MIT.
The research team sees lots of uses for this new algorithm, including replacing materials such as petroleum with products that have a far smaller carbon footprint. Another idea posited was new protein-inspired food coatings which could keep produce fresh for longer.
“In addition to their natural role in living cells, proteins are increasingly playing a key role in technological applications ranging from biologic drugs to functional materials. In this context, a key challenge is to design protein sequences with desired properties suitable for specific applications. Generative machine-learning approaches, including ones leveraging diffusion models, have recently emerged as powerful tools in this space,” said Tuomas Knowles, professor of physical chemistry and biophysics at Cambridge University.
While consumer-focused generative systems have got most of the online buzz, there is a large movement of pharmaceutical AI research which is now using generative tools to quickly create proteins and drugs at a much faster and cheaper rate. This new form of research is still contained mostly in academia, but we expect in the next few years large pharmaceutical companies take advantage of the lower costs and faster discovery offered by these generative systems.
“In the biomedical industry, you might not want a protein that is completely unknown because then you don’t know its properties. But in some applications, you might want a brand-new protein that is similar to one found in nature, but does something different. We can generate a spectrum with these models, which we control by tuning certain knobs,” said Buehler.
Alongside the rush to add generative systems to almost all forms of AI research, the release of AlphaFold by DeepMind has inspired a huge amount of academic research into protein structures and using AI to create novel materials at a fraction of the usual cost. While MIT does not mention AlphaFold in the press release, it is quite clear that the open distribution of this database has pushed forward academic research into these pharmaceutical problems.