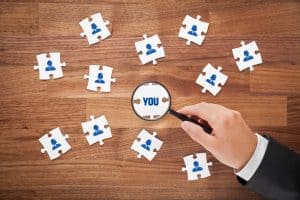
Real-time personalization allows businesses to build next-level relationships with their customers.
Business is all about relationships. From awareness to retention, what draws customers in and persuades them to stay is how a company makes them feel. And nothing makes a customer feel more special than when a company remembers who they are and gives them what they want. Personalizing service at a local ice cream shop requires remembering names, faces, and regular orders. Personalization at scale requires a whole different set of skills.
Even in 2005, when I wrote “The Perfect Message at the Perfect Moment” in the Harvard Business Review, I was obsessed with personalization at scale. I’ve been focused on making this process scalable, dynamic, and effective. But don’t take my word for it– a McKinsey report predicts that personalization at scale has the potential to create $1.7 trillion to $3 trillion in new value across almost every industry. However, capturing this value will require mastering the technologies and addressing the organizational disconnects that underlie massive personalization undertakings. This article will outline the challenges of building personalization at scale, along with the number one way businesses are choosing to overcome them.
Real-time personalization
For every business, it pays to know your customers. This goes deeper than knowing each person’s history; to treat a customer how they want to be treated every moment, businesses need real-time insights.
We’ll illustrate the effect real-time personalization insights can have by using a retail website. Knowing what someone put in their shopping cart should change the recommendations they are given immediately. For example, if someone has previously ordered exercise shorts but currently has a pair of stylish black boots and a dressy blouse in their shopping cart, it doesn’t make sense to recommend running sneakers! They are more likely to buy work slacks, as they are likely shopping for professional clothing at the moment.
Benefits from real-time customer insights also apply in customer service, like in a telecommunications company. Let’s say a customer’s internet router is disconnected and reconnected twice, then the number linked to the account comes into a call center. If the representative is able to see the customer’s data in real time, they can start out knowing what’s wrong and immediately apologize and help troubleshoot, instead of needing an already frustrated customer to convey their predicament.
These are two minor examples of how real-time personalization can help companies, employees, and customers. In order to get there, companies have to overcome a few different challenges.
Data Challenges associated with personalization
“The difference between personalization and personalization at scale is data.”
Personalization depends on a profound understanding of the customer: their history, preferences, needs, choices, and demographics. This can be parsed together with data from disparate sources: purchasing history, web cookies, interaction with customer service and advertisements, and more.
However, if all of this data is produced and stored in disconnected systems, applications can’t easily access it all to build a cohesive picture. Data must come together in a single location for reliable customer data to best deliver relevancy for personalization applications. To accomplish this, you need to enable data unification from owned channels, like your transaction data (i.e., customer, call center, and engagement data) with data from paid media (i.e., social, advertising, and third-party data).
Building a strong data foundation means centralizing data. However, it takes more than putting all the data in one place to data architecture.
Technology challenges
Machine learning is the key to personalization, as this technology can take in vast amounts of data and adapt based on what is learned much more quickly, accurately, and affordably than people can. However, machine learning models don’t learn well from raw data, any more than you or I could gain meaning from a long string of numbers in an excel sheet.
Cleaning, resampling, and transforming this data is necessary for it to be useful for machine learning models. Clean data has to be transformed into features that machine learning models can learn from in order to come to conclusions.
The process of taking this raw data and transforming it into features so models can understand it is called feature engineering, and this is one of the most time-consuming parts of the machine learning process. Not only is it time-consuming, but it’s also monotonous and prone to errors.
Automating the feature engineering process saves time and energy for data scientists, which means they can get models up and running faster. Even though technology and data are the main roadblocks to personalization, organizational structures are also important to consider when implementing enterprise-wide personalization efforts.
Organizational challenges
Data siloing is the enemy of personalization because it prevents all the key pieces from coming together to reveal a complete picture of the customer within a reasonable time frame.
Traditionally, the people who can manage large volumes of data and encode it in machine learning models are sitting in IT departments with people who share their technical skillset. They are one step removed from the business and less intimately acquainted with the most important business inputs and outcomes. The models they make reflect this, often collecting data for a variable that is not as predictive as another.
The AI or data science team simply does not have enough knowledge about the business or the applications that will deploy the models to optimize production operations that deliver business outcomes. To overcome this, the secret sauce to successful personalization implementation is diversity. Data scientists need to work side by side with people who know the customers and the application from inception to completion.
To solve this problem, you need to build cross-functional teams across the organization. A “personalization SWAT team” composed of marketers, data scientists, and salespeople will be the most effective in knowing what data to collect, what features to build, and what outcomes to optimize when building personalization applications.
While the technical and organizational roadblocks to personalization at scale may seem daunting, a new technology is emerging on the market that allows businesses to personalize customer care like never before. A feature store provides a central location for all the data related to every customer and every interaction they have ever had.
A feature store is a central repository that stores features, data lineage, and metadata associated with all the machine learning models in a company. In essence, it is a single source of truth for all of the data science work within one organization. Being able to share and re-use features boosts data science productivity by cutting down duplicate work and making it easy for data engineers, data scientists, and ML engineers to collaborate, overall making each machine learning model cheaper and easier to produce.
With feature stores automating and centralizing key parts of the machine learning process, data scientists can focus on collaborating with different departments that can define key business inputs and outcomes so companies will receive the ROI they’ve been waiting for. Implementing an integrated personalization-focused organizational structure will revolutionize the returns machine learning models are able to offer to employees and shareholders alike.
Conclusion
When building relationships, it’s not enough to know what a customer has done in the past. In order to best support them, you’ll need to predict a customer’s future wants and needs and deliver content specifically tailored to them. By centralizing customer data, automating feature engineering, and enabling collaboration across a company, a feature store allows you to implement personalization across your company like never before.